Which Immigrants Succeed? Simple Facts to Guide Better Policy
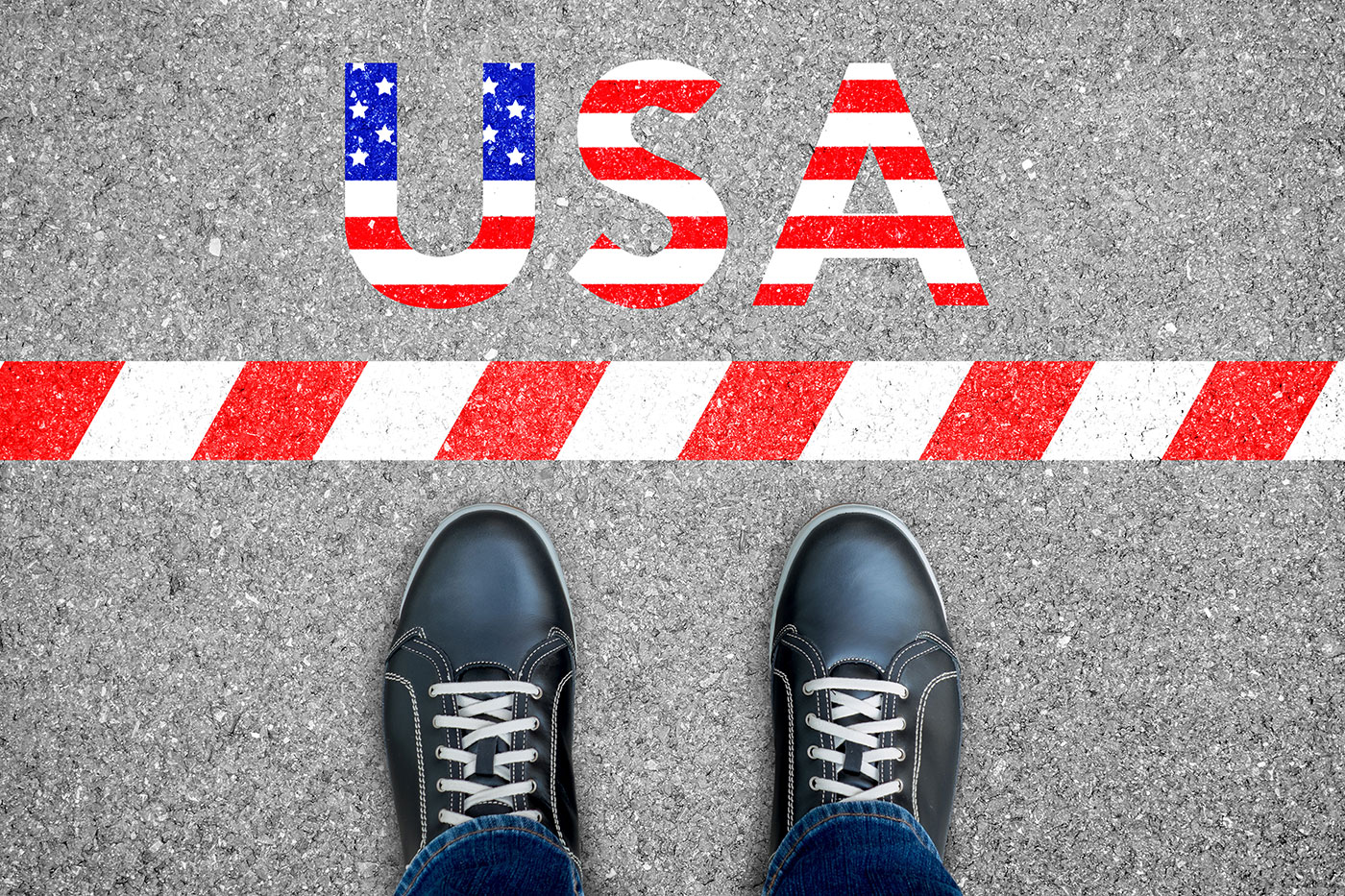
Executive Summary
While other developed nations go out of their way to recruit and admit high-skilled immigrants, the U.S. places much more emphasis on family ties. This report demonstrates, in a simple and straightforward way, the price of taking this approach.
Using the Census Bureau’s American Community Survey to track immigrants across their prime earning years (my sample includes those who were born between 1968 and 1972, came to the U.S. as adults, and were interviewed in the 2000s and 2010s) I show that higher-skilled immigrants—those with higher levels of education, more elite occupations, and better English skills—perform enormously better on numerous metrics, including income.
To take just one example, in 2019, immigrants in my sample with no high school diploma—who were then about 50 years old—had mean personal earnings of about $26,000. Those with a high school diploma but no college made a bit more, about $33,000. Those with a B.A. came in above $73,000, while those with post-graduate education earned roughly $130,000. These numbers reflect the combined effects of labor-force participation, unemployment, wages and salaries, and institutionalization.
High-skilled immigrants benefit the country in many ways—they pay more in taxes, use fewer government benefits, spur more innovation and job creation, and compete for jobs with the richest Americans rather than the poorest. In order to better reap these benefits, several reforms to current immigration policy should be pursued. These range from simple tweaks that would make high-skilled immigration easier to a complete overhaul of the legal immigration system that places less weight on family connections and more on skills.
Introduction
American immigration policy has been at a standstill for decades. Some on the right would like to drastically curtail both legal and illegal immigration, and some on the left would like to enact the most permissive system possible. A politically viable middle ground has been hard to find.
But the status quo fails to achieve a very basic goal: getting the most out of the immigrants we admit, whatever total number we choose. Many other developed countries aggressively pursue this goal through a skills-based “point system,” but in the U.S., far more immigrants receive permanent residency through family ties than on the basis of valuable skills. Further, thanks to a porous border and lackluster interior enforcement, many immigrants were not “chosen” at all.
Among the roughly 25 million “prime-working-age” (i.e., 25–54) foreign-born individuals living in the U.S. in 2019, 23% lacked a high school diploma. The respective number for the native-born was just 7%. At the same time, immigrants were also slightly more likely to have post-graduate education—16% vs. 13%—thanks to some elements of the current system that do attract and admit the highly educated. We don’t categorically refuse to pay attention to immigrants’ skills; we just don’t make it much of a priority.
The purpose of this report is to lay out, in stark and simple terms, how easy it would be to select immigrants with drastically improved economic and social outcomes. I show that immigrants with higher levels of education, more elite occupations, and better English fluency perform enormously better on numerous metrics, including income. And higher earnings mean more taxes paid, fewer government benefits used, more innovation and job creation, and competition for jobs with the richest Americans rather than the poorest.
The direction of these results will surprise no one, but the scale of the differences—outcomes are often several times better for certain subsets of immigrants—highlights the consequences of admitting newcomers with in-demand skill sets.
In the aggregate, immigrants’ earnings are predictable based on the skills they come with, and the current system does not make enough use of this information. In addition to documenting immense differences in outcomes across different types of immigrants I also explain the policies that created this situation and options for reform.
The Policy Status Quo
There are several key elements to our current immigration system.
For legal immigration, the key document is the “green card,” which grants permanent residency. Setting aside the years 2020 and 2021—when levels fell due to the pandemic, before rebounding[1]—the U.S. has recently granted roughly a million of these cards per year.
As of 2019,[2] roughly two-thirds of green cards were given out on the basis of family ties; more than a third of those went to relatives who were not spouses or minor children of citizens or permanent residents. (There is no cap at all for “immediate relatives” of U.S. citizens, including spouses, minor children, and even parents. Additional visas are available—albeit limited in number and often with long waits—for other relatives, including adult children and siblings of citizens, as well as spouses
and children of current green-card holders.)[3] Another 10% of green cards went to refugees and asylees, while 4% were awarded through the Diversity Visa Lottery—which admits immigrants literally at random, so long as they have a high school diploma and come from a country that otherwise sends few migrants to the U.S.
Just 14% of green cards were awarded through employment-based preferences, and about half these green cards went to dependents of workers rather than workers themselves.
Beyond green cards, the U.S. also grants tens of millions of temporary visas each year, and while these are largely for short-term visitors such as tourists and business travelers, several types of visas often lead to permanent residence. Some are “dual intent” visas expressly designed for this purpose. Indeed, typically, about half of those granted green cards are already in the country on other visas.[4]
Of particular note are the F-1 visa, given to students, and the H-1B, given to high-skilled workers, often graduates of U.S. colleges transferring from an F-1. The H-1B has a cap of 85,000 (20,000 reserved for those with advanced degrees), but certain employers, including universities, are exempt from the cap, so total issuances can be twice that number.[5] In cases subject to the cap, the H-1B is awarded by lottery rather than being given to the most deserving or highest-paid candidates, and H-1B recipients are somewhat limited in their ability to change employers.
Finally, many immigrants come to the country illegally, either by crossing the border surreptitiously or by getting a temporary visa and simply not leaving when they are supposed to. By its very nature, illegal immigration is difficult to measure precisely, but mainstream estimates place the current number of immigrants in the country illegally at around 10 million—a figure that represents about a fifth to a quarter of the total immigrant population. Owing to the U.S. policy of birthright citizenship, any children born to illegal immigrants on U.S. soil are automatically considered American citizens.
The question is, how do various types of immigrants differ in their outcomes? And what might we expect to happen if we were to change the mix of immigrants we admit?
The Basics of the Data
I measure immigrant outcomes using the American Community Survey (ACS), which has collected data on more than a million Americans every year since 2001 (and several million in more recent years).[6] Specifically, I downloaded the ACS microdata through IPUMS,[7] a University of Minnesota–based project that cleans the data and calculates certain useful additional variables.
The ACS randomly samples addresses each year and collects data about the individuals living in each housing unit—it doesn’t repeatedly question the same people. However, one can still use the data to “follow” broad demographic segments of the population. In this case, I’ll be looking at immigrants born between 1968 and 1972 and tracking their performance from 2001 through 2019.[8]
The immigrants are limited to those who entered the country in 2001 or earlier (to keep the cohort stable over time) as adults (as our focus is on how to select adult immigrants, not the kids who come with them).[9] In total, I have more than 300,000 observations.[10] Since 2006, the ACS has sampled about 1% of the population,[11] so each observation represents roughly 100 American residents, on average, in the year the person was surveyed.
In other words, we will see how a small cohort of people—early-to-mid–Generation Xers—fared as they made their way through their thirties and forties. This setup takes full advantage of the two-decade span of the ACS, and it focuses on prime-earning years that typically come well after education but well before retirement.
There are a few limitations to this approach. For one thing, since individuals sometimes die or leave the U.S., the populations we are “following” are not precisely the same from year to year. Immigrants, unsurprisingly, are a bit more likely to leave than natives are; the immigrant population represented in the sample shrinks by about 10% over the course of the data set. Another limitation is that the ACS excluded individuals in institutional group quarters (such as prisons and mental-health facilities) until 2006; this is a tiny share of the overall population but slightly frustrates efforts to measure trends in institutionalization itself. Further, in part because of the uncapped green-card preference for parents of U.S. citizens, immigration has increasingly skewed older in recent years,[12] and people who come at older ages are not included in my sample at all.
Additionally, much of my analysis focuses on sorting individuals by traits such as their education and occupation. Most people in the age range covered in my data have already finished their education and settled into a field, so shifts in these categories over time are minimized.
Figure 1 illustrates this fact for the major sorting variables that we’ll be discussing. Some individuals continue to acquire new degrees in their thirties or later, for example, but in my immigrant sample, this tendency is modest. (The same analysis run for native-born Americans reveals much stronger shifts, interestingly.) Perhaps the most notable trend is the improvement in immigrants’ English skills over time.
Figure 1.
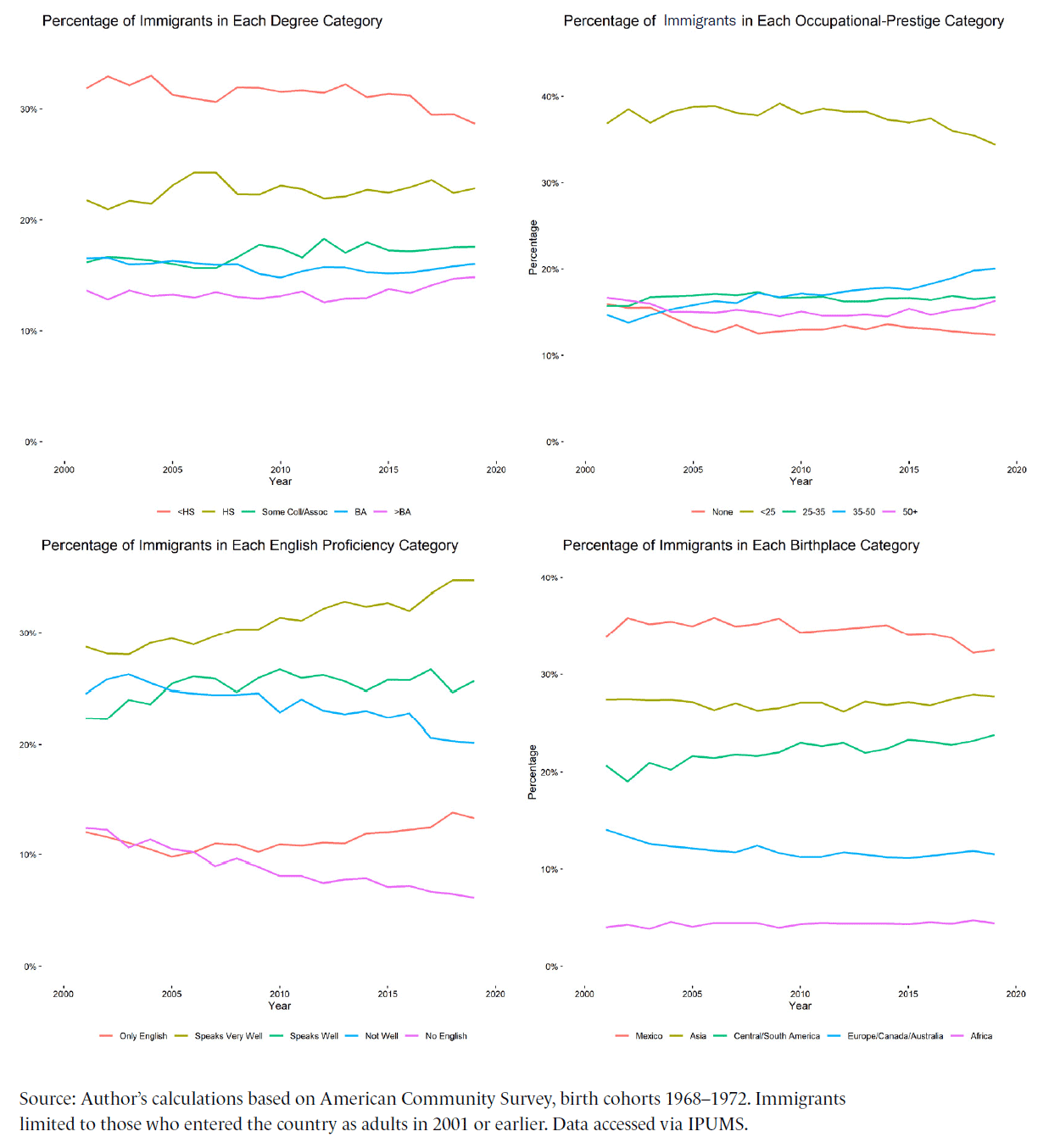
Differences by Education
Education levels are the simplest and most common proxy for skill levels. To be sure, there are plenty of skilled laborers who far outearn the college graduates who end up working at Starbucks, but education levels remain a strong general indicator of a worker’s value on the job market.
Many employers see a college degree as valuable in itself—perhaps a sign that the degree-holder learned something important in school, or at least a signal that the person has the basic levels of intelligence, conscientiousness, and conformity needed to make it through a degree program. And some of the highest-paying occupations—doctor, lawyer, etc.—often legally require high levels of education, regardless of the preferences of any specific employer.
It is therefore common sense that if we select immigrants who have (or are coming to the U.S. to earn) higher-level degrees, we will end up with better economic performance from those immigrants years later. But how dramatic are the differences?
Figure 2 shows the mean[13] personal earned incomes of individuals in each education category over time, including both wage and business income as well as those who report zero earnings or losses in the averages. Those with post-graduate degrees were earning six figures, on average, by the end of the 2010s, when they were about 50 years old. These figures are given in contemporary dollars—incomes in, say, 2005 represent actual incomes in 2005 and are not adjusted for inflation. This way, readers may compare the numbers below with their own earnings and earning trajectories during the same years (though bear in mind that some of the increase over time is simply due to inflation).
Figure 2.
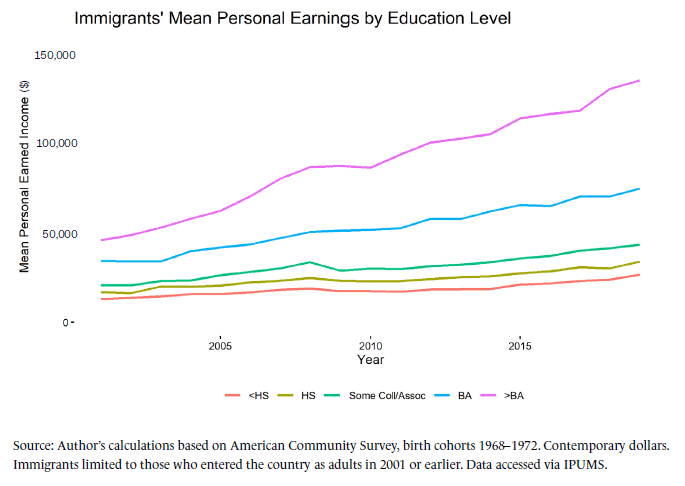
A limitation of these data is that very high incomes are “top-coded” to protect privacy. (From 2003 on, the average earnings of the top 0.5% within each state are calculated and assigned to everyone in the group.) Also note that the stagnant incomes for high school dropouts are overstated, both because many of the more capable dropouts in the early 2000s later left the category by earning degrees, and because those in prisons and mental-health facilities are not included until 2006.
Statistical nuances aside, the central message is clear: immigrants with higher levels of education earn more, sometimes much more, than those with lower levels of education.
Also worth noting is that while the census’s broad education categories pertain to levels of schooling rather than the content of the education or the quality of the school, it does also collect information about field of study. Among immigrants with a B.A., for instance, mean personal earnings in 2019 were far higher among those who studied “Computer and Information Sciences” (about $100,000) than among those who studied “Communications” (about half of that). Survey respondents are given more than 35 fields as options (some of the numbers have wide confidence intervals, though, so I’ll spare readers the full chart).
Figures 3 and 4 show two other major economic indicators: labor-force participation, meaning whether people have jobs or are at least looking for jobs and unemployment, which means lacking a job despite wanting one.
For labor-force participation, I disaggregated the results by sex, since women are far more likely than men to leave the labor force to take care of children in this age range. Immigrant men (unlike native-born men) have extremely high participation regardless of their education. Women are more likely to remain in the labor force if they have higher education levels.
Figure 3.
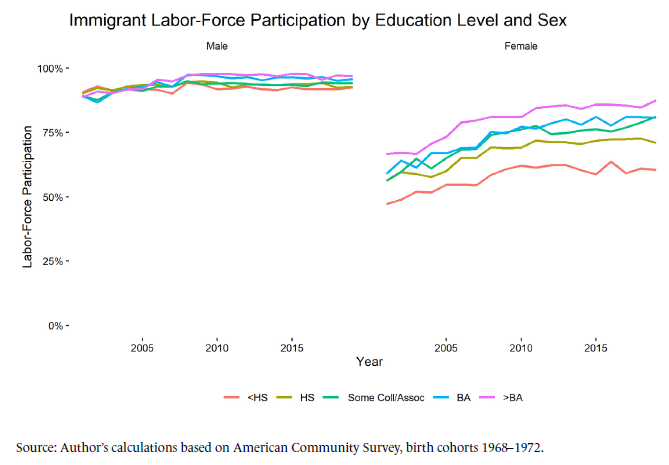
Among individuals who are in the labor force, here are the unemployment rates by education. Clearly, those with higher levels of education are more likely to have a job if they want one.
Figure 4.
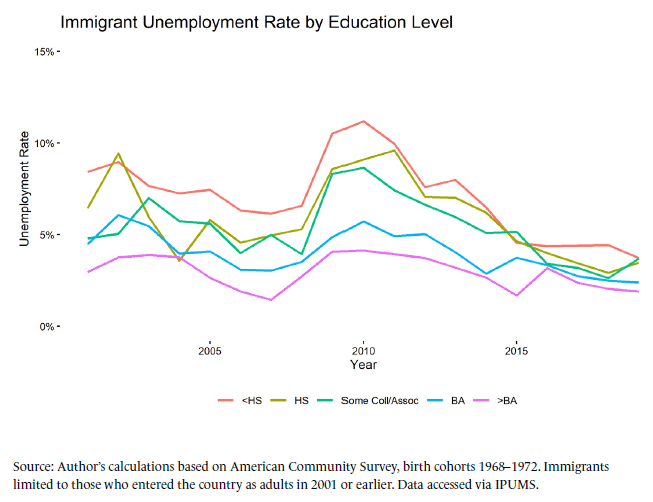
A major controversy within the immigration debate is the extent to which immigrants use safety-net benefits and what restrictions should be placed on such benefits. A recent Manhattan Institute poll showed that Americans place high value on admitting immigrants who do not use welfare, with 35% choosing it as the most important factor, beating out willingness to assimilate and ability to fill jobs, among other choices.[14]
The ACS allows us to track some benefits, but these data have limits. Benefits receipt is self-reported (like most items in any survey) and thus is likely underreported.[15] Also, respondents are asked whether anyone in the entire household uses benefits, so it does not specifically capture the individual’s own receipt. This is important because there is controversy about how to count benefits given to the U.S.-born (and therefore native U.S. citizens) minor children of immigrants.[16]
With these limitations in mind, Figure 5 provides the numbers on how many individuals in my sample live in households that report using food stamps, which are among the most widely used safety-net benefits. Clearly, those with higher levels of education are far less likely to live in households that receive food stamps.
Figure 5.
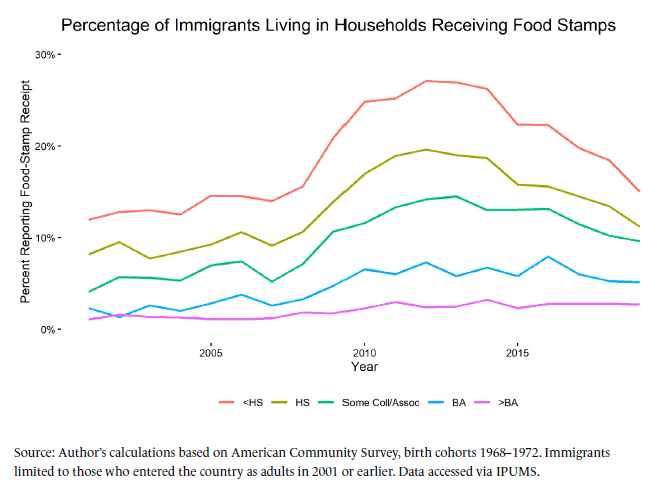
Noneconomic Outcomes
Another key social outcome available in the ACS is institutionalization—specifically, whether the respondent is living in institutional group quarters such as a prison or mental-health facility. As noted above the ACS did not include these locations until 2006; further, the ACS does not record which type of institution respondents are living in.
Figure 6 shows the available data, once again disaggregated by sex, given the higher crime and incarceration rates of men. Institutionalization is rarer among immigrants than among natives—and indeed, the ACS contains zero institutionalized immigrants in some of these combinations of year, sex, and educational attainment—but rates are minimized among those with higher education levels.
Figure 6.
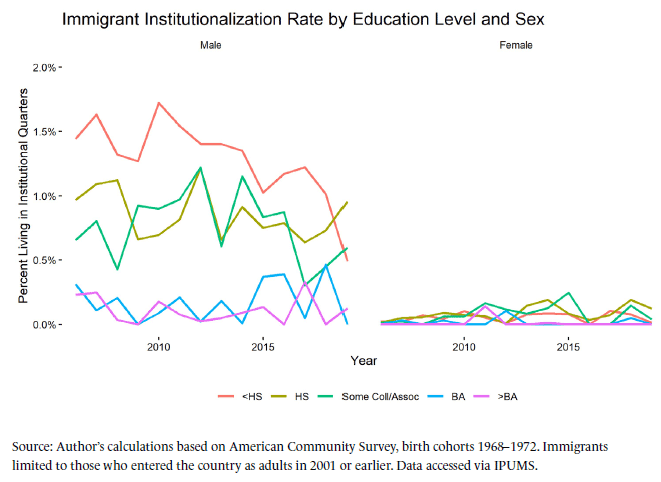
Fertility is yet another key social indicator—and immigration is often floated as a curative for low native fecundity, though the immigration rates required to make a dent in the overall birthrate would need to be unrealistically large[17]—but it’s captured somewhat unevenly in the ACS. The data do not show how many children respondents have in total only if they gave birth in the past year and how many of their children are living with them currently. In my sample, which begins after many women have given birth, one reasonable approach is to measure how many children are living with each woman every year (as women are usually most likely to get custody of children in the event of a breakup, and children generally don’t move out until adulthood). This gives a sense not only of how many children these women had in total but also of when they gave birth and when the kids started moving out.
Figure 7.
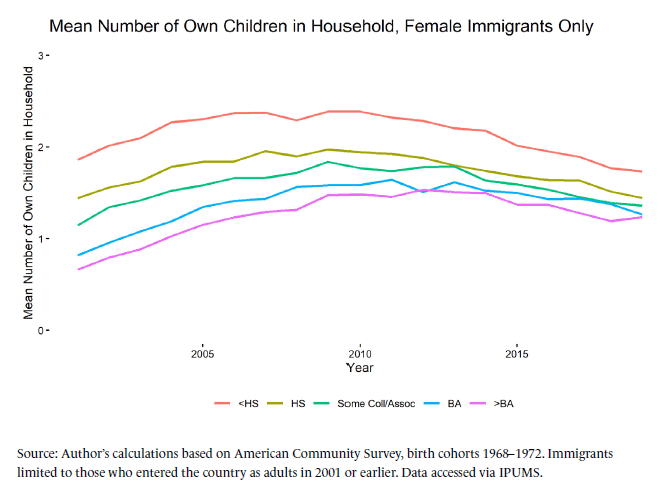
As shown in Figure 7, fertility is generally a bit higher for women with lower education levels, which presents a key trade-off of selecting immigrants based on high skills.
A related social outcome, however, is unmarried childbearing and child-rearing, which is generally associated with worse outcomes for children, though there is some debate over the extent to which this statistical relationship is causal.[18] Among mothers living with at least one child, Figure 8 shows the portion who are currently married (and not separated). Married child-rearing is strongly linked to education levels.
Figure 8.
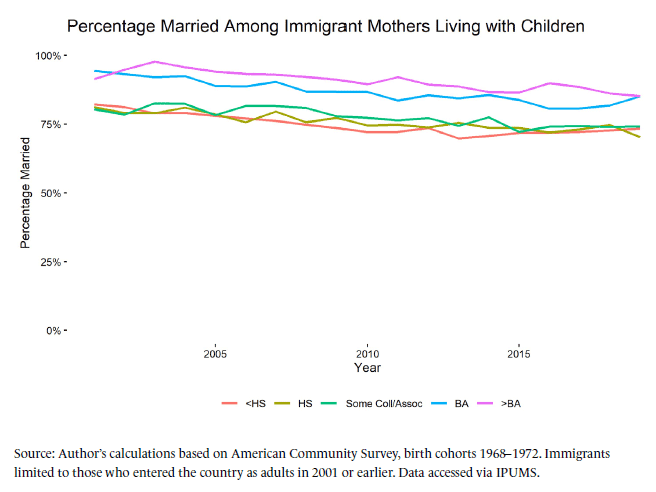
The Roles of Occupation, English, and Birthplace
Education is a handy proxy for skill levels, but it’s hardly the only variable we might consider (or already do consider) when setting immigration policy. Among any group of people with the same broad education level, there will be immense variation in outcomes. One paper suggests this is especially true for immigrants.[19]
Occupation is an especially promising avenue: Rather than relying on education to indicate marketable skills, we can—and to a limited extent do, through employment-based visas—simply admit people who are ready to do specific jobs. We could also select those with strong English proficiency, which enables easy communication with employers, customers, neighbors, and so on. We might also look for performance differences among immigrants from different parts of the world.
In theory, it would be possible to redo the entire previous analysis with each of these traits in place of education—charting occupation vs. unemployment, birthplace vs. fertility, and all the rest. In the interests of space, however, I’ll simply stick with personal earnings as the outcome of interest.
The ACS has, if anything, too much information about occupation, with too many different codes to analyze individually (and even more codes denoting the industry the respondent works in). Fortunately, there are simple, if perfect, systems for classifying occupations by their overall prestige, factoring in the typical earnings and educational requirements.
In my analysis, I employ the Hauser and Warren Socioeconomic Index (HWSEI), a system created in 1990 and included with the IPUMS version of the ACS data,[20] and divide scores into a few groupings (Figure 9). Occupations scoring below 25 include, for example, farm workers, child-care workers, janitors, and construction workers. Between 25 and 35 are secretaries, salespeople, and hairdressers; from 35 to 50 are insurance underwriters and many types of managers. Above 50 sit such lofty professions as legislator, engineer, doctor, and lawyer. Those with no HWSEI classification are excluded from the chart; they generally have not worked in five years and report little or no earned income.
Of course, it’s somewhat tautological to point out that people in higher-status jobs earn more, given that status is partly a function of typical earnings. But that simply highlights how easy and straightforward it would be to pick candidates for immigration based on criteria that predict six-figure incomes. It is entirely possible, after all, to allow people into the country for the specific purpose of performing a given occupation known to pay well.
Figure 9.
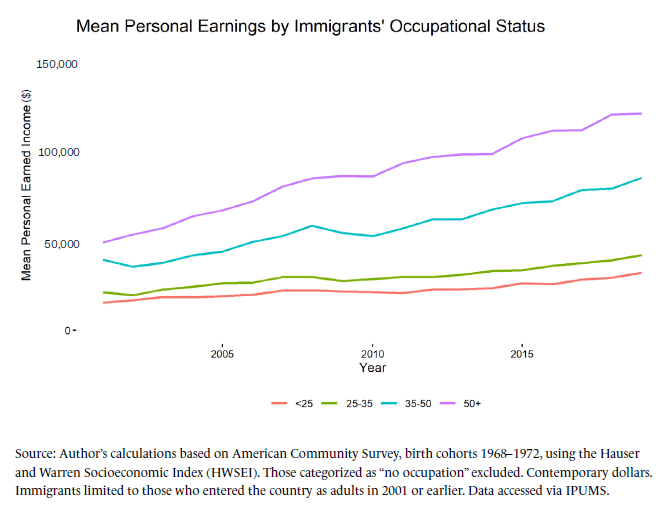
We could also pay more attention to English proficiency, which correlates strongly with earnings (Figure 10).
Figure 10.
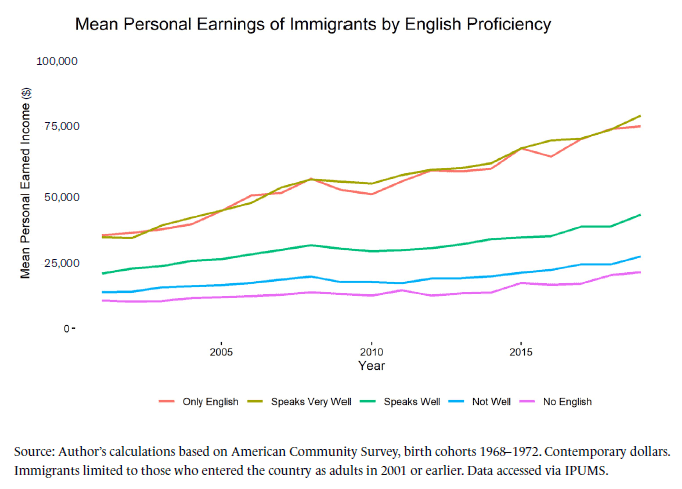
Lastly, there’s birthplace. This is a fraught topic, as birthplace correlates with traits we would not want to discriminate on the basis of (most notably race), but I include it for two reasons. First, the current system already discriminates based on birthplace via the “per-country caps” placed on green cards (no country may receive more than 7% of employment- and family-based cards, with no adjustment for the population of the country or the number of people who want to immigrate), as well as the Diversity Visa Lottery, which hands out green cards through a lottery limited to people from countries that otherwise send few immigrants. And second, some have argued—for instance, the economist Garett Jones in his recent book The Culture Transplant[21]—that immigrants bring some cultural features of their old countries with them, and immigration policy should take account of this.
As with occupations, I collapsed the many birthplaces available in the ACS down to a handful of categories, including the country with the most immigrants during this period (Mexico), and several other groups (roughly continent, but with the Anglosphere merged into Europe).
Figure 11.
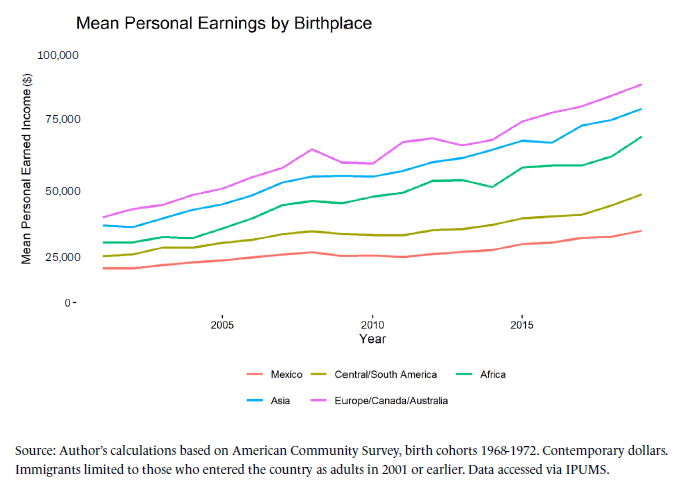
There are substantial differences based on birthplace (Figure 11), but those differences may simply reflect that some places send immigrants with more education, and so on, than others. Indeed, all the relationships we’ve seen thus far may be confounded with one another. We can investigate further by combining these traits into a single statistical model.
A Combined Model
By now, it should be clear that there are many outcomes we may want to maximize with our immigration policy. For the purpose of this report, however, I will stick with personal earnings.
This poses some thorny technical issues: earnings are “skewed”(with a small fraction of the population earning far above the average)—a problem that can often be solved by using a logarithmic scale. However, because earnings numbers can also contain zeros or negative numbers representing losses, it is mathematically impossible to log these numbers. There’s no ideal solution—every existing possibility either distorts the data in some way or is difficult to interpret, but a trick called the “inverse hyperbolic sine” (IHS) transformation can produce easily interpretable results, and different approaches I tried led to basically similar findings.[22]
I ran a model that included the various traits we’ve looked at up to this point, plus the number of years each immigrant has been in the U.S., to capture one of the advantages of bringing talented people here at younger ages—they can establish their connections and careers earlier and thus tend to make more than other immigrants of the same age, education level, and so on—though variation here is limited by the fact that everyone in the sample had arrived by 2001 as an adult. To account for basic population demographics, inflation, and so on, I also included categorical variables for sex, age, and calendar year.
Most variables have one category chosen as the “reference,” a sort of baseline, and the results of the other categories can roughly be interpreted as a percentage difference versus the reference. (The coefficients have been exponentiated, 1 subtracted, and the result multiplied by 100[23] to make this as straightforward as possible.) The exception is the number of years in the U.S., where the result indicates the effect of each additional year.
One complication of a model like this is especially worth noting: the occupational-prestige variable tends to “steal” the influence of the others. For example, English proficiency or an associate degree not only improves earnings for people within occupations but can also increase earnings by getting people into better occupations. Including occupation itself in the model, however, absorbs this effect. Similarly, the other traits might also affect long-term non-work, which is absorbed by the occupation variable’s “no occupation” level; virtually all those in that category report zero earnings.
More practically speaking, in cases where an immigrant’s occupation is known, we might be able to observe his current earnings or job offer directly, so this variable might be of less use. Therefore, I ran the model both with and without occupational prestige (Table 1).
Table 1.
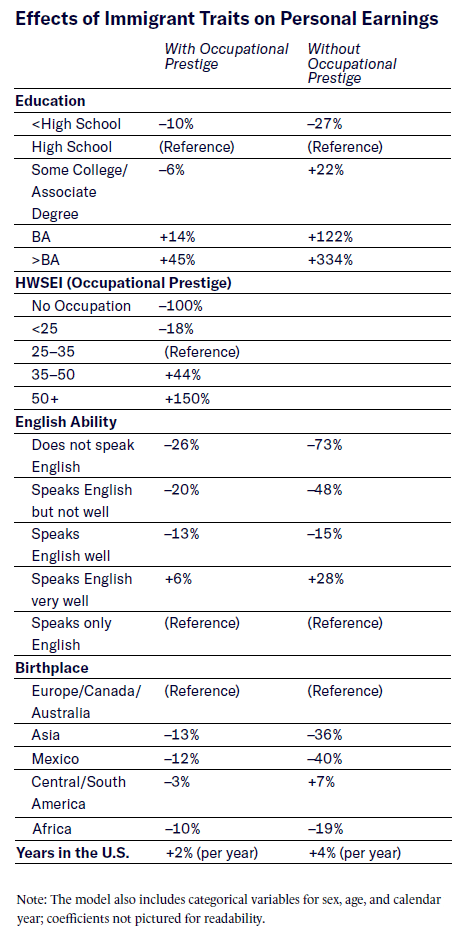
Effects of Immigrant Traits on Personal Earnings
The first model shows that occupation is an extremely powerful variable and should have outsize influence on immigration decisions when available; the second shows that high-level degrees and English proficiency are both very powerful otherwise. An unexpected finding is that those who’ve learned to speak English “very well” somewhat outperform English-only speakers, though this was not evident in some other approaches to modeling these data and likely reflects quirks of the IHS transformation and/or variables not in the model (such as field of study).
Since the models include several traits simultaneously, the results can tell us, for example, whether education still predicts earnings after accounting for things like occupational prestige. (This is not the same as showing whether these variables directly cause earnings, but when it comes to selecting the immigrants most likely to do well, predicting is all we really need to do.) Birthplace does improve predictions after accounting for the other variables, but the differences are more subtle and less consistent and could reasonably be ignored, out of concerns for discriminating based on illegitimate criteria. This is especially true if, as I propose in a later section, we make efforts to collect more finely grained data on which types of education and employment most reliably lead to higher earnings.
Note, relatedly, that real-life trade-offs will involve changing several variables at once: Admitting people with better occupations, for instance, will almost certainly entail admitting people with higher levels of education.
General Policy Implications
From the foregoing, it is abundantly clear that—on average—the economic fortunes of adult immigrants are predictable. Those with high levels of education, the ability to perform in-demand jobs, and proficiency in English overwhelmingly tend to succeed. Such effects might wash out over the course of several generations of assimilation and intermarriage,[24] but in several generations, we’ll all be dead.
In the short- and medium-term, America’s economy and tax coffers undeniably benefit from some immigrants far more than others. A 2017 report from the National Academies used a wide variety of strategies to estimate the 75-year fiscal impact of a new immigrant, aged 25–64, with varying degrees of educational attainment. (Older immigrants were consistently a net drain on public finances, while younger ones were simply assigned the education level of their parents, making the results harder to interpret.) But no matter the set of assumptions involved—for instance, whether immigrants were assumed to increase the cost of “public goods” such as the military, and whether future Congresses were assumed to exercise fiscal restraint or spend freely—those with a high school diploma or less tended to cost the government more than they paid in, with the opposite being true for those with a college degree or higher. Both the losses and the gains frequently numbered in the hundreds of thousands of dollars per immigrant.[25]
Starting with these facts—as well as a fundamental assumption that America’s immigration policy exists, at least in large part, to advance the national interest by improving its economy—one might interpret these findings in two ways.
First, one might argue that—despite its many benefits—immigration risks social instability, backlash, and at least some negative economic consequences for Americans who compete with the newcomers for jobs[26] and housing[27] (or pay taxes to fund safety-net benefits); surveys show only minority support for increasing or decreasing overall immigration levels from what they are currently.[28] Through this lens, an obvious possibility is to keep our overall immigration level roughly the same while rebalancing the system to place far greater weight on skills. If that works and the public’s attitudes toward immigration become more positive, we can then return to the question of overall levels.
Alternatively, one might point out that high-skilled immigrants are essentially a sure bet. On average, these individuals will innovate, create jobs, and pay lots of taxes—and to the extent that they compete with natives, they’ll be competing with the best-off among us and thus reducing inequality. Regardless of what’s happening with the rest of the system, then, we should push to expand high-skilled immigration, and we are leaving free money on the table if we fail to do so.
Of course, this approach is essentially a wager that higher overall immigration levels won’t cause social problems despite the concerns Americans express in polls, but it avoids the political fight inherent in any attempt to cut low-skilled immigration.
I’ll be focusing on the first approach here, which aligns with my own views, but for an intelligent, comprehensive proposal tracking more closely with the second approach, I recommend a recent Hamilton Project report,“Renewing America, Revamping Immigration,” written by Jennifer Hunt.[29]
Specific Reforms to Consider
To maintain overall immigration levels while placing greater emphasis on skills, a deep redesign of the immigration system is called for. Family-based green cards should be restricted to spouses and minor children of U.S. citizens and permanent residents, and the Diversity Visa Lottery should be abolished—U.S. residency should never be given out literally at random. To improve the pipeline of green-card applicants already in the country, the H-1B lottery should also be replaced by a system in which the visas are given to those with the highest earnings or simply auctioned off to the companies that want immigrant workers the most. To the extent that policymakers wish to address the legal and illegal immigration systems together, they might also consider improved border security and interior enforcement.
In general, immigrants should be selected through a point system. The primary focus should be to select immigrants who (A) will likely do well given their employment prospects, degrees, and English proficiency and (B) fall into that precious young-adult age range where they can establish connections and careers early and then live their full prime earning years in the U.S. before retiring. Family connections to individuals already here (siblings and so on) can be taken into account but should be given significantly less weight. The model results presented above give a basic sense of how a point system might weight these various factors, though they understate the value of younger immigrants. (In addition to earning more than otherwise similar immigrants, which is what the model measures, those who come younger simply work and pay taxes for a greater number of years before they retire.)
Recent graduates with high-quality degrees and good jobs, or good job offers if they are applying from outside the U.S., are an especially promising target. And while the system should let in spouses and minor children along with high-skilled immigrants, it might also include spouses’ own education and so on as a consideration, rather than relying solely on the fact that social outcomes tend to be correlated within families.
I am not proposing a complete end to low-skilled immigration. Political necessity will dictate reserving some slots, or setting up a guest-worker program, for industries reliant on such labor, especially if illegal-immigration reforms succeed in reducing unlawful entry. (Agricultural labor is a particular concern here; even setting aside politics, in the absence of immigration, the country would likely turn to automation or imports before paying American workers enough to do this work.) Refugee and asylee admissions should continue as well, for humanitarian reasons. Reductions to lower-skilled, family-based immigration could create room for expanding admissions for these most sympathetic cases. The trade-off is that, if overall levels remain the same, any slots allocated to these purposes are slots that cannot be used for expanding high-skilled immigration.
Several other smaller-scale reforms are also worth mentioning.
For one thing, the U.S. government should do far more to carefully track immigrants’ outcomes, not only by education level and field of study but also in terms of what degree program and college they attended, what companies they work at, and what positions they work in. For natives, recent years have seen great advances in tracking of outcomes associated with the degree programs offered by each U.S. college.[30] We should invest in similar efforts focused on immigrants, including those who graduated from schools in other countries. Green-card preferences should be given to degree programs that reliably produce high-performing graduates and companies with a history of attracting and lavishly compensating top talent.
Such a system also has predecessors in other nations, including Canada’s Longitudinal Immigration Database and Australia’s Continuous Survey of Australia’s Migrants. These systems allow governments to assess outcomes, improve the immigration system, and present good data to the public regarding what current policy accomplishes.[31] Another predecessor is the New Immigrant Survey here in the U.S., with initial interviews in 2003 and follow-ups with the same individuals later that decade, but this has not been repeated.[32]
My Manhattan Institute colleague Daniel Di Martino, in a recent pair of reports, has proposed some more incremental reforms that are worth noting.[33] These include granting work authorization to the dependents of high-skilled workers (who are often barred from working under current policy), relaxing regulations that dramatically increase wait times, and allowing applicants to pay for expedited processing.
Conclusion
That there are many traits that powerfully predict high economic performance, but U.S. immigration policy does relatively little to select for these traits. As the extremes of the immigration debate emphasize either open borders or extreme restrictions, a middle ground is to embrace the power of skills to advance the national interest.
About the Author
Robert VerBruggen is a fellow at the Manhattan Institute where he provides policy research, writes for City Journal, and contributes to special projects and initiatives in the president’s office. Having held roles as deputy managing editor of National Review, managing editor of The American Conservative, editor at RealClearPolicy, and assistant book editor at the Washington Times, VerBruggen writes on a wide array of issues including economic policy, public finance, health care, education, family policy, cancel culture, and public safety. VerBruggen was a Phillips Foundation Journalism Fellow in 2009 and a 2005 winner of the Chicago Headline Club Peter Lisagor Award. He holds a B.A. in journalism and political science from Northwestern University.
Endnotes
Photo: mantinov/iStock
Are you interested in supporting the Manhattan Institute’s public-interest research and journalism? As a 501(c)(3) nonprofit, donations in support of MI and its scholars’ work are fully tax-deductible as provided by law (EIN #13-2912529).