Is Wikipedia Politically Biased?
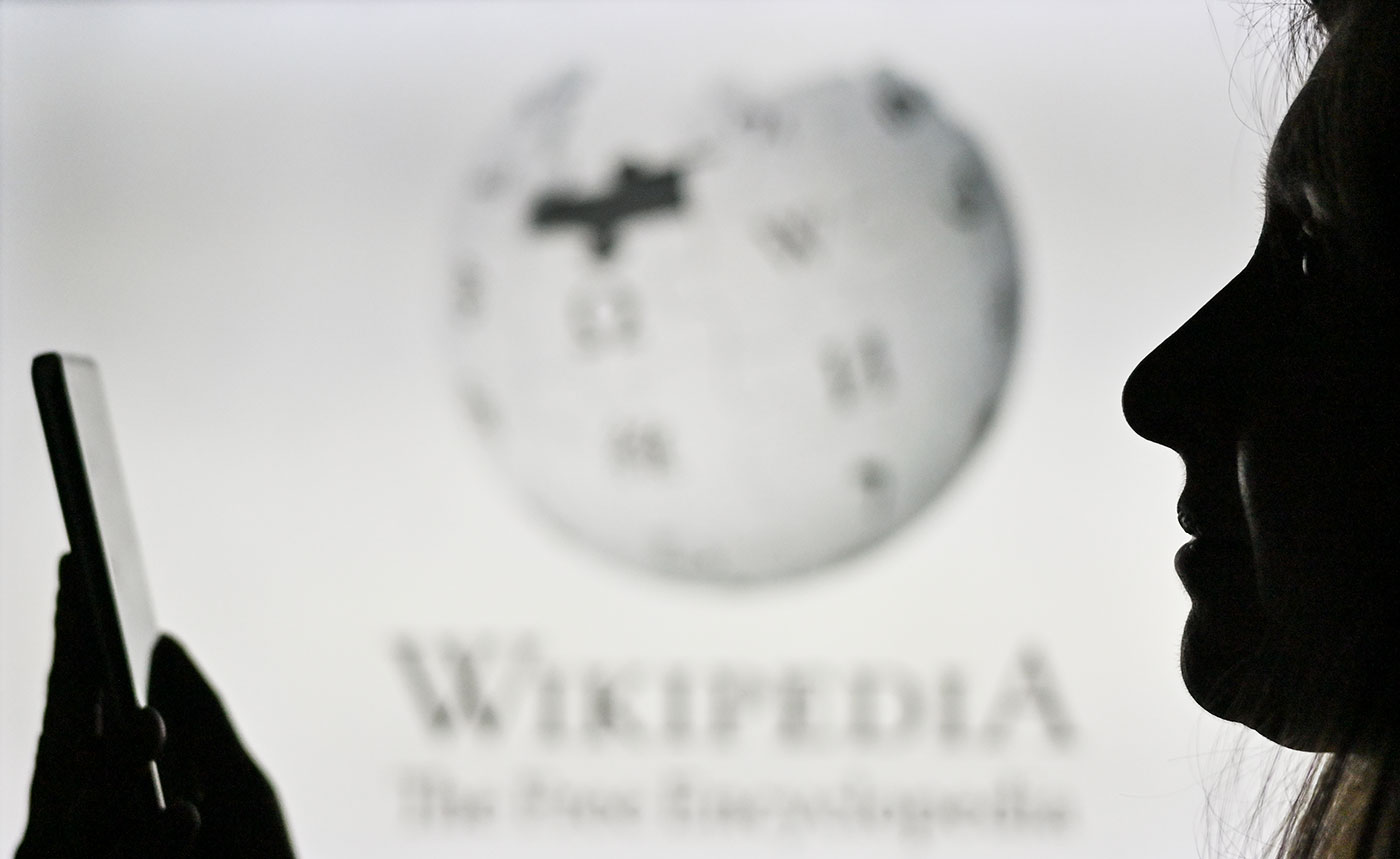
Executive Summary
- This work aims to determine whether there is evidence of political bias in English-language Wikipedia articles.
- Wikipedia is one of the most popular domains on the World Wide Web, with hundreds of millions of unique users per month. Wikipedia content is also routinely employed in the training of Large Language Models (LLMs).
- To study political bias in Wikipedia content, we analyze the sentiment (positive, neutral, or negative) with which a set of target terms (N=1,628) with political connotations (e.g., names of recent U.S. presidents, U.S. congressmembers, U.S. Supreme Court justices, or prime ministers of Western countries) are used in Wikipedia articles.
- We do not cherry-pick the set of terms to be included in the analysis but rather use publicly available preexisting lists of terms from Wikipedia and other sources.
- We find a mild to moderate tendency in Wikipedia articles to associate public figures ideologically aligned right-of-center with more negative sentiment than public figures ideologically aligned left-of-center.
- These prevailing associations are apparent for names of recent U.S. presidents, U.S. Supreme Court justices, U.S. senators, U.S. House of Representatives congressmembers, U.S. state governors, Western countries’ prime ministers, and prominent U.S.-based journalists and media organizations.
- This trend is common but not ubiquitous. We find no evidence of it in the sentiment with which names of U.K. MPs and U.S.-based think tanks are used in Wikipedia articles.
- We also find prevailing associations of negative emotions (e.g., anger and disgust) with right-leaning public figures; and positive emotions (e.g., joy) with left-leaning public figures.
- These trends constitute suggestive evidence of political bias embedded in Wikipedia articles.
- We find some of the aforementioned political associations embedded in Wikipedia articles popping up in OpenAI’s language models. This is suggestive of the potential for biases in Wikipedia content percolating into widely used AI systems.Wikipedia’s neutral point of view (NPOV) policy aims for articles in Wikipedia to be written in an impartial and unbiased tone. Our results suggest that Wikipedia’s NPOV policy is not achieving its stated goal of political-viewpoint neutrality in Wikipedia articles.
- This report highlights areas where Wikipedia can improve in how it presents political information. Nonetheless, we want to acknowledge Wikipedia’s significant and valuable role as a public resource. We hope this work inspires efforts to uphold and strengthen Wikipedia’s principles of neutrality and impartiality.
Introduction
Wikipedia.org is the seventh-most visited domain on the World Wide Web, amassing more than 4 billion visits per month across its extensive collection of more than 60 million articles in 329 languages.[1] Launched on January 15, 2001, by Jimmy Wales and Larry Sanger, Wikipedia has evolved over the past two decades into an indispensable information resource for millions of users worldwide. Given Wikipedia’s immense reach and influence, the accuracy and neutrality of its content are of paramount importance.
In recent years, Wikipedia’s significance has grown beyond its direct human readership, as its content is routinely employed in the training of Large Language Models (LLMs) such as ChatGPT.[2] Consequently, any biases present in Wikipedia’s content may be absorbed into the parameters of contemporary AI systems, potentially perpetuating and amplifying these biases.
Smart Policy, Straight to You
Don't miss the newsletters from MI and City Journal
Since its inception, Wikipedia has been committed to providing content that is both accurate and free from biases. This commitment is underscored by policies such as verifiability, which mandates that all information on Wikipedia be sourced from reputable, independent sources known for their factual accuracy and commitment to fact-checking.[3] Additionally, Wikipedia has a neutral point of view (NPOV) policy, which requires that articles are “written with a tone that provides an unbiased, accurate, and proportionate representation of all positions included in the article.”[4]
Many of Wikipedia’s articles cover noncontroversial topics and are supported by a wealth of objective information from several sources, so achieving NPOV is ostensibly straightforward. However, attaining NPOV is far more challenging for articles on political or value-laden topics, on which the debates are often subjective, intractable, or controversial, and sources are often challenging to verify conclusively.
The question of political bias in Wikipedia has been the subject of previous scholarly investigations. In 2012, a pioneering study using a sample of more than 20,000 English-language Wikipedia entries on U.S. political topics provided the first evidence that political articles on the site generally leaned pro-Democratic.[5] The authors compared the frequency with which Wikipedia articles mentioned terms favored by congressional Democrats (e.g., estate tax, Iraq war) versus terms favored by Republicans (e.g., death tax, war on terror) and found that the former appeared more frequently. This study was replicated by its authors in 2018, yielding similar results.[6] Other research focusing on Wikipedia’s arbitration process in editorially disputed articles has documented biases in judgments about sources and in enforcement, in a manner that shows institutional favoritism toward left-of-center viewpoints.[7]
Other research has questioned whether, and in which direction, Wikipedia’s content is biased. Some reports suggest that at least some political content in Wikipedia is biased against left-of-center politicians.[8] There is also research suggesting that Wikipedia’s content is mostly accurate and on par with the quality of articles from Encyclopaedia Britannica.[9]
Anecdotally, Larry Sanger, one of Wikipedia’s cofounders, has said publicly that he believes that the site has a high degree of political bias in favor of a left-leaning, liberal, or “establishment” perspective. Sanger has accused Wikipedia of abandoning its neutrality policy and, consequently, he regards Wikipedia as unreliable.[10]
The goal of this report is to complement the literature on Wikipedia’s political bias by using a novel methodology—computational content analysis using modern LLMs for content annotation—to assess quantitatively whether there is political bias in Wikipedia’s content. Specifically, we computationally assess the sentiment and emotional tone associated with politically charged terms—those referring to politically aligned public figures and institutions—within Wikipedia articles.
We first gather a set of target terms (N=1,628) with political connotations (e.g., names of recent U.S. presidents, U.S. congressmembers, U.S. Supreme Court justices, or prime ministers of Western countries) from external sources. We then identify all mentions in English-language Wikipedia articles of those terms.
We then extract the paragraphs in which those terms occur to provide the context in which the target terms are used and feed a random sample of those text snippets to an LLM (OpenAI’s gpt-3.5-turbo), which annotates the sentiment/emotion with which the target term is used in the snippet. To our knowledge, this is the first analysis of political bias in Wikipedia content using modern LLMs for annotation of sentiment/emotion.
In general, we find that Wikipedia articles tend to associate right-of-center public figures with somewhat more negative sentiment than left-of-center public figures; this trend can be seen in mentions of U.S. presidents, Supreme Court justices, congressmembers, state governors, leaders of Western countries, and prominent U.S.-based journalists and media organizations. We also find prevailing associations of negative emotions (e.g., anger and disgust) with right-leaning public figures and positive emotions (e.g., joy) with left-leaning public figures. In some categories of terms, such as the names of U.K. MPs and U.S.-based think tanks, we find no evidence of a difference in sentiment.
Our results suggest that Wikipedia is not living up to its stated neutral–point–of–view policy. This is concerning because we find evidence of some of Wikipedia’s prevailing sentiment associations for politically aligned public figures also popping up in OpenAI’s language models, which suggests that the political bias that we identify on the site may be percolating into widely used AI systems.
Results
Before turning to the common political terms analyzed, we first validate our methodology (for specific methodological details, see Appendix) by applying it to terms that are widely accepted to have either negative or positive connotations. As Figure 1 shows, we find that terms that nearly all would agree have positive associations—such as prosperity, compassion, Martin Luther King, and Nelson Mandela—are generally used in Wikipedia articles with positive sentiment; and terms with widely accepted negative connotations—such as disease, corruption, and Joseph Goebbels—are generally used with mostly negative sentiment.
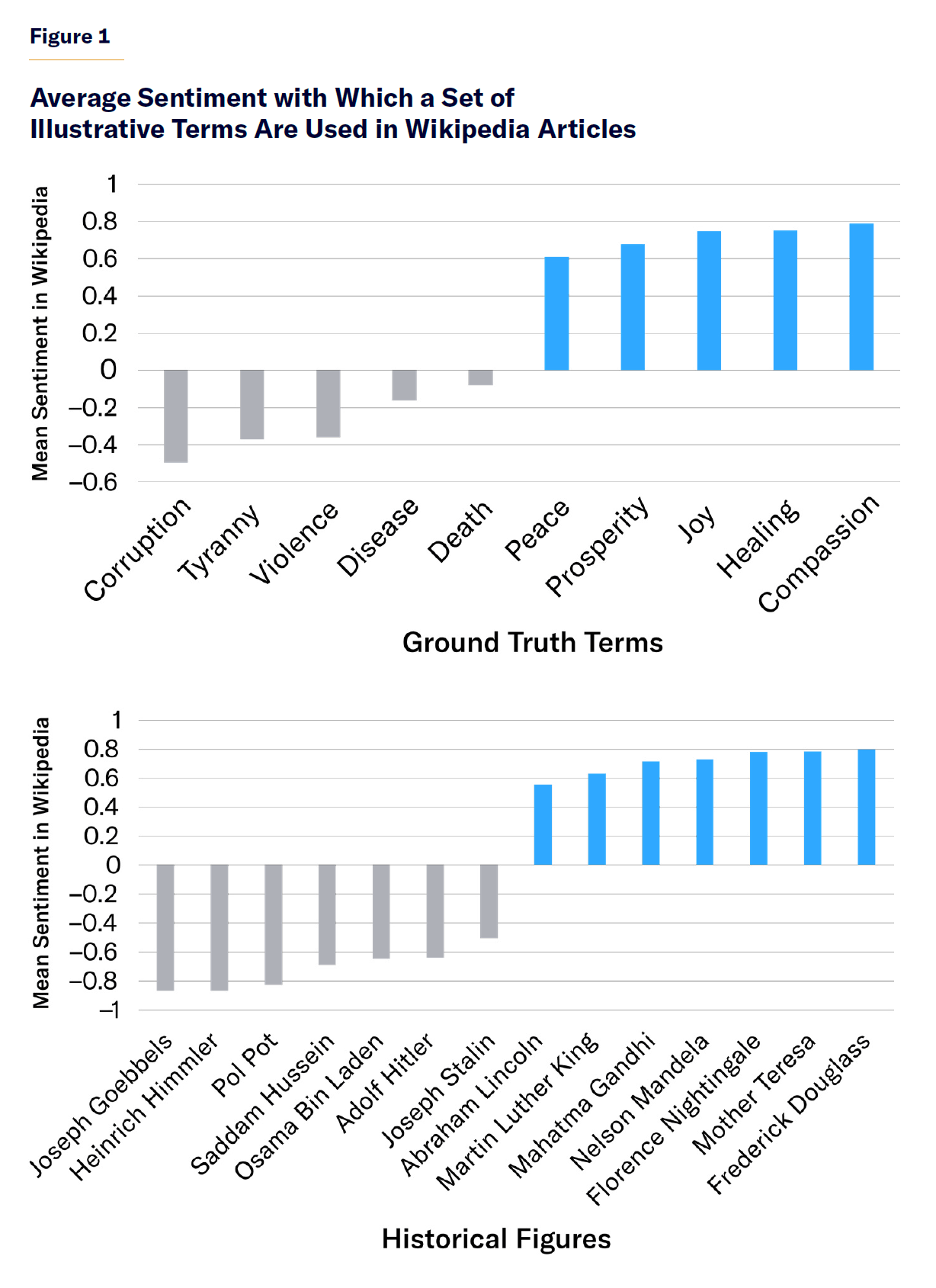
Next, we analyze how a set of terms that denote political orientation and political extremism are used in Wikipedia articles (Figure 2). The sample set of terms is small, but there is a mild tendency for terms associated with a right-leaning political orientation to be used with more negative sentiment than similar terms for a left-leaning political orientation.
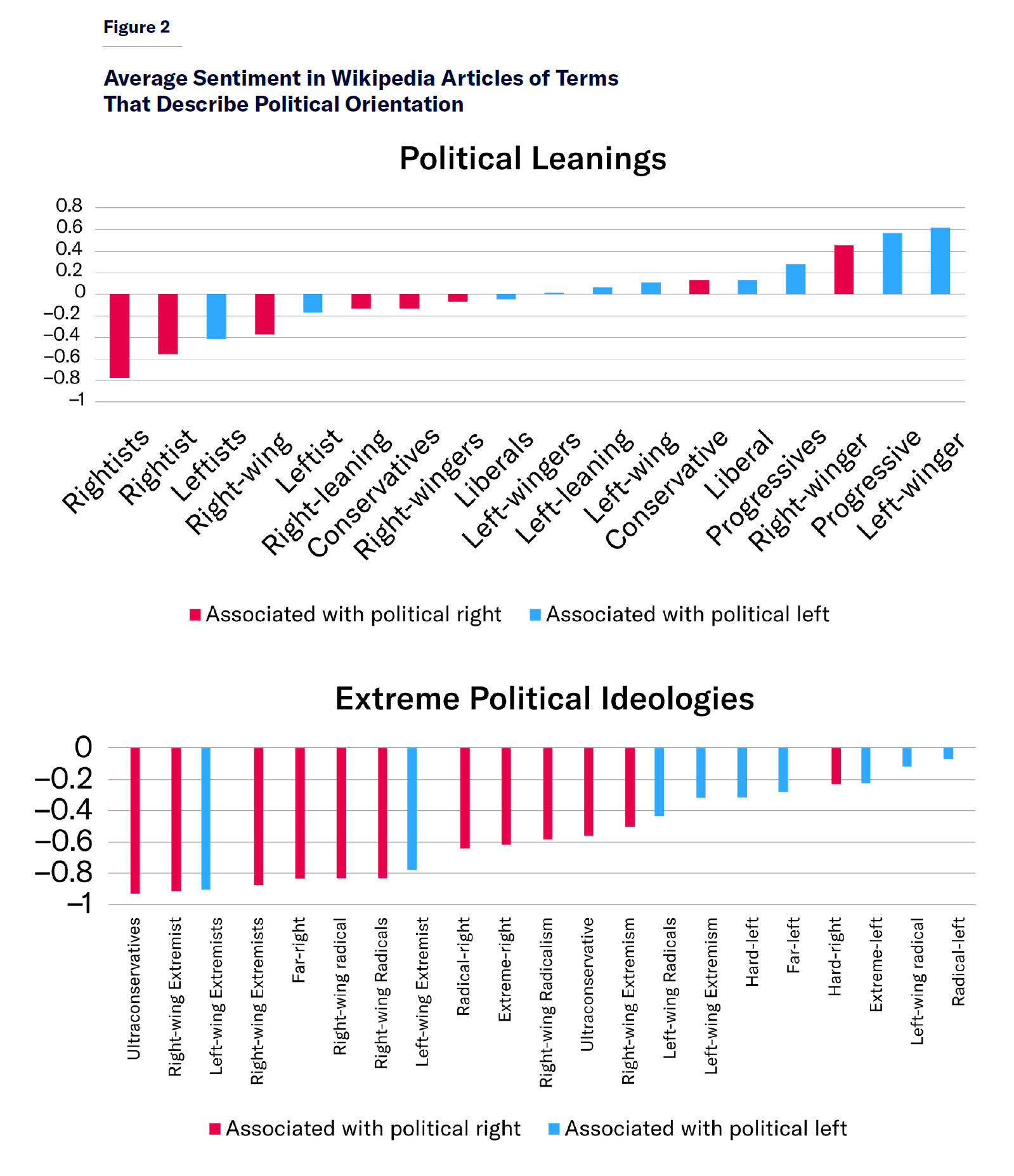
Some of the most important results in this report are shown in Figure 3, which displays the average sentiment with which recent U.S. presidents, members of the 117th Congress, and state governors (as of 2022) are used in Wikipedia articles. There is a clear tendency for the names of prominent left-leaning U.S. politicians to be used with more positive sentiment than their right-leaning counterparts. Unfortunately, this method cannot account for instances where different people have the same title (e.g., President Bush Senior and President Bush Junior, or Republican Senator Kennedy of Louisiana and the late Democratic Senator Kennedy of Massachusetts); but these cases are relatively rare, so their overall effect on the aggregate results should be marginal.
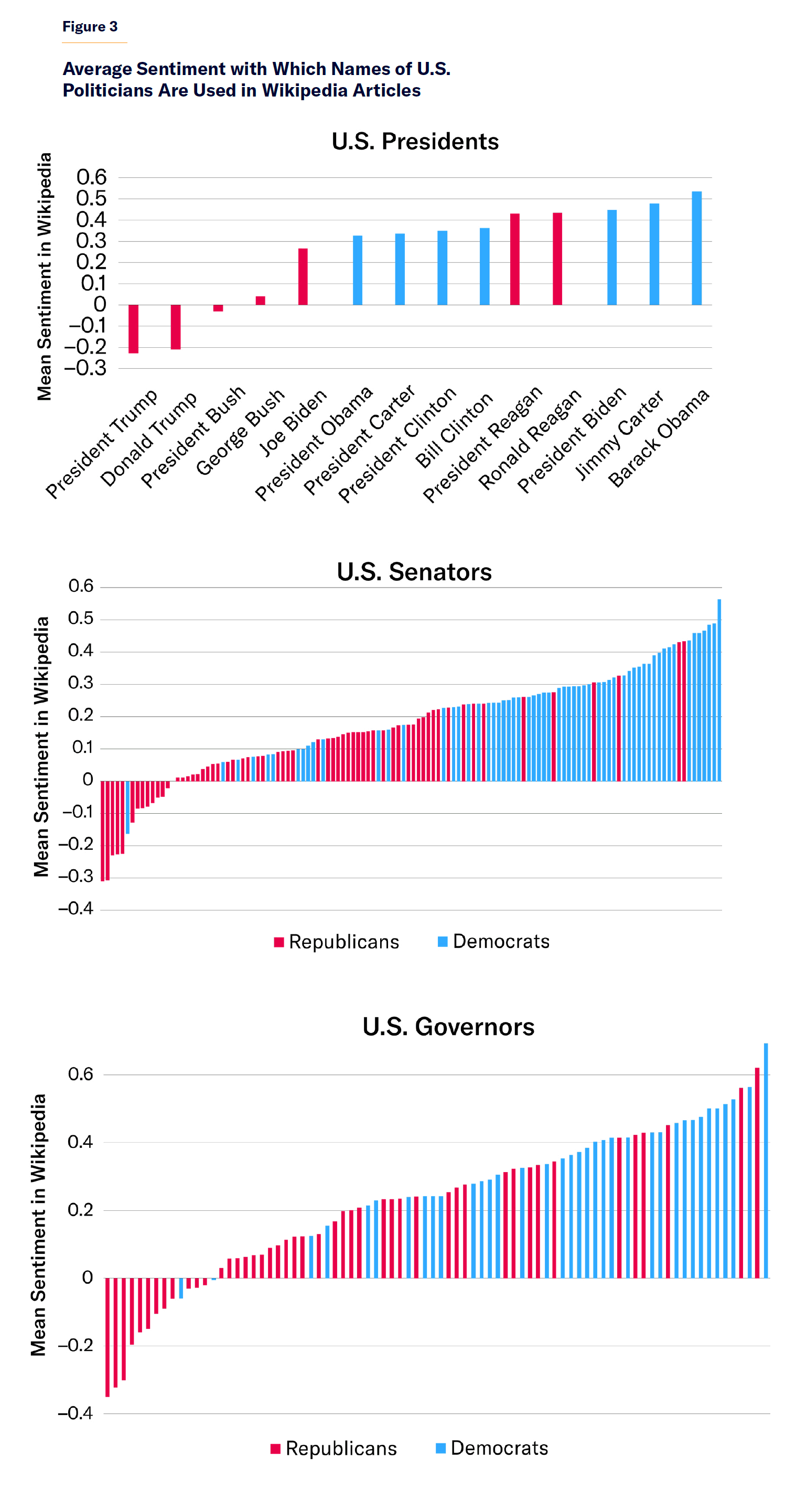
U.S. Governors Note: Each person is represented in the plots with two terms (i.e. Ron DeSantis and Governor DeSantis). Only terms with at least 20 occurrences in Wikipedia are displayed. Full data available here.
Average sentiment with which recent U.S. presidents, senators, congressmembers and state governors are used in Wikipedia articles. Full data available here.
The trend toward more positive sentiment for left-wing figures in Wikipedia articles is not limited to elected officials. The same can be seen for the names of U.S. Supreme Court justices and prominent U.S.-based journalists (Figure 4).
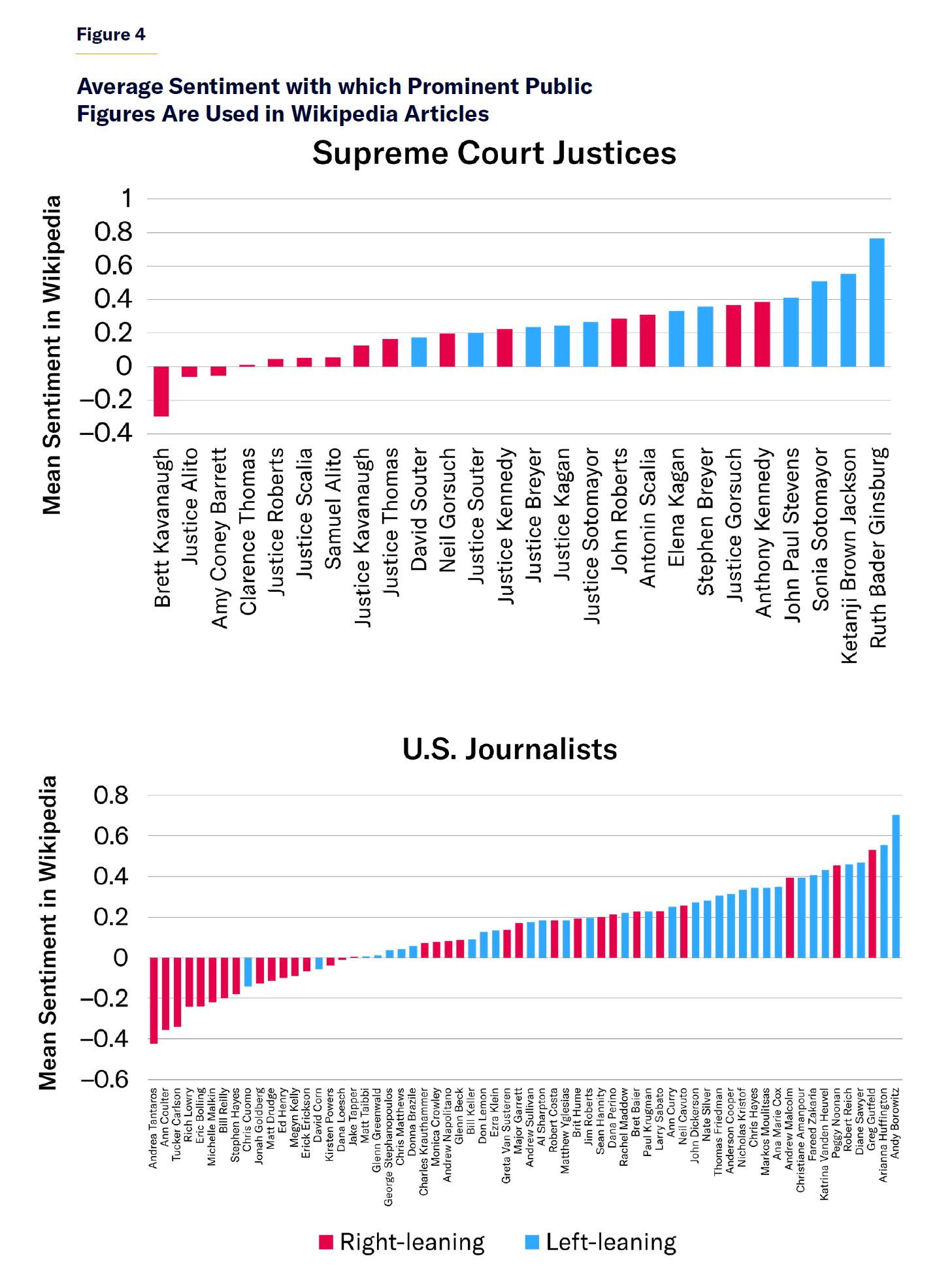
Nor is this phenomenon limited to public figures in the United States. A similar trend can be seen in how prime ministers from prominent Western countries (since the year 2000) are described in Wikipedia articles. This pattern, however, does not hold for Wikipedia mentions of U.K. MPs. (Figure 5). (Note that the dominant red color in the bottom of Figure 5 is due to the larger number of Conservative MPs than Labour MPs in the U.K. Parliament because of the 2019 U.K. general election.) The disparity of sentiment associations in Wikipedia articles between U.S. Congressmembers and U.K. MPs based on their political affiliation may be due in part to the higher level of polarization in the U.S. compared to the U.K. Wikipedia entries about non-political public figures sharing the same name with political figures could also be playing a confounding role in this regard.
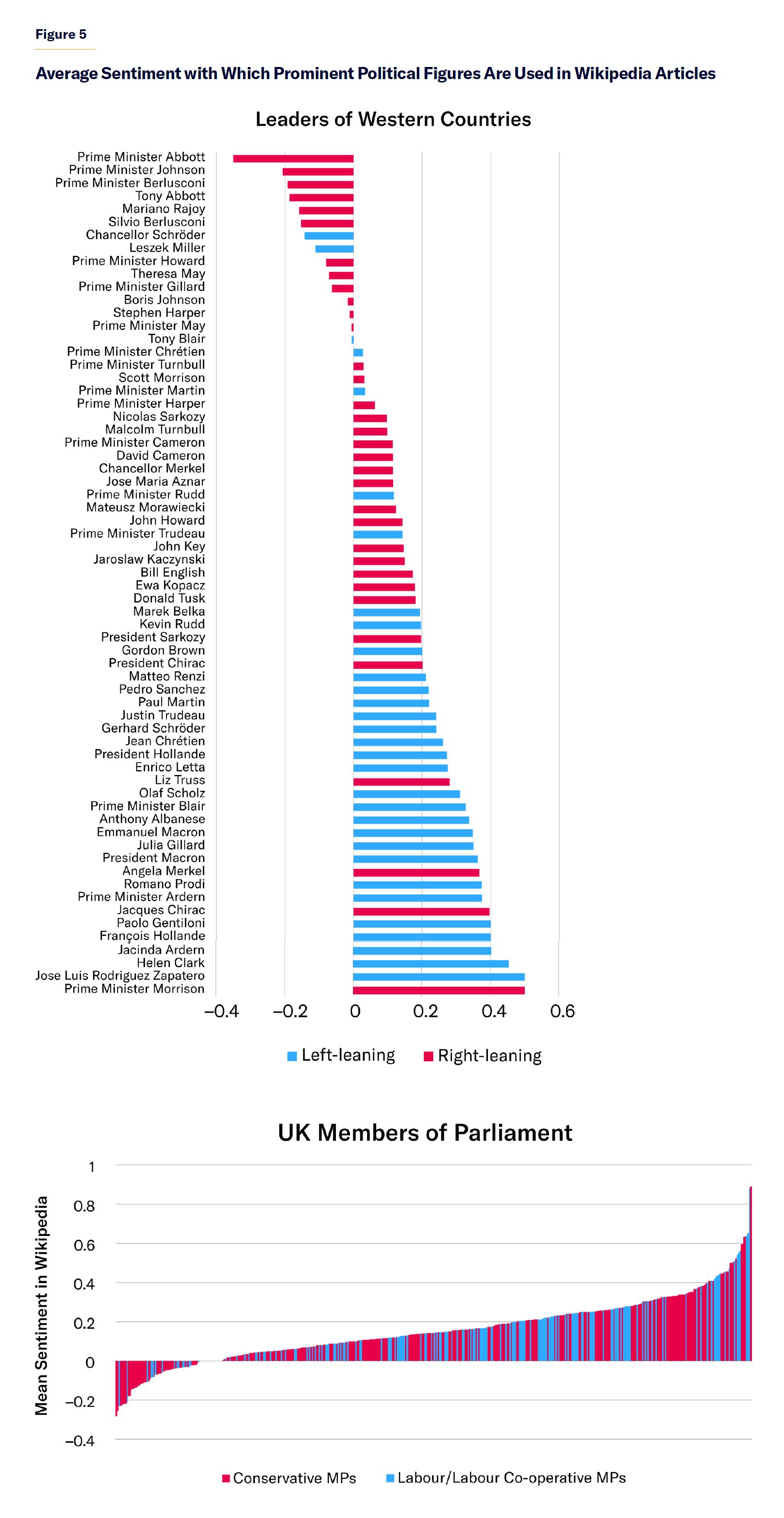
The asymmetry in sentiment associations on Wikipedia entries between right and left public figures can also be seen for influential news media organizations. Figure 6 shows that Wikipedia articles tend to refer to left-leaning news media institutions with more positive sentiment than right-leaning news media organizations. However, there is no difference in sentiment in Wikipedia’s descriptions of right-leaning vs. left-leaning U.S.-based think tanks. This may be because think tanks do not elicit polarizing emotional responses as strongly as do media organizations or politically aligned journalists.
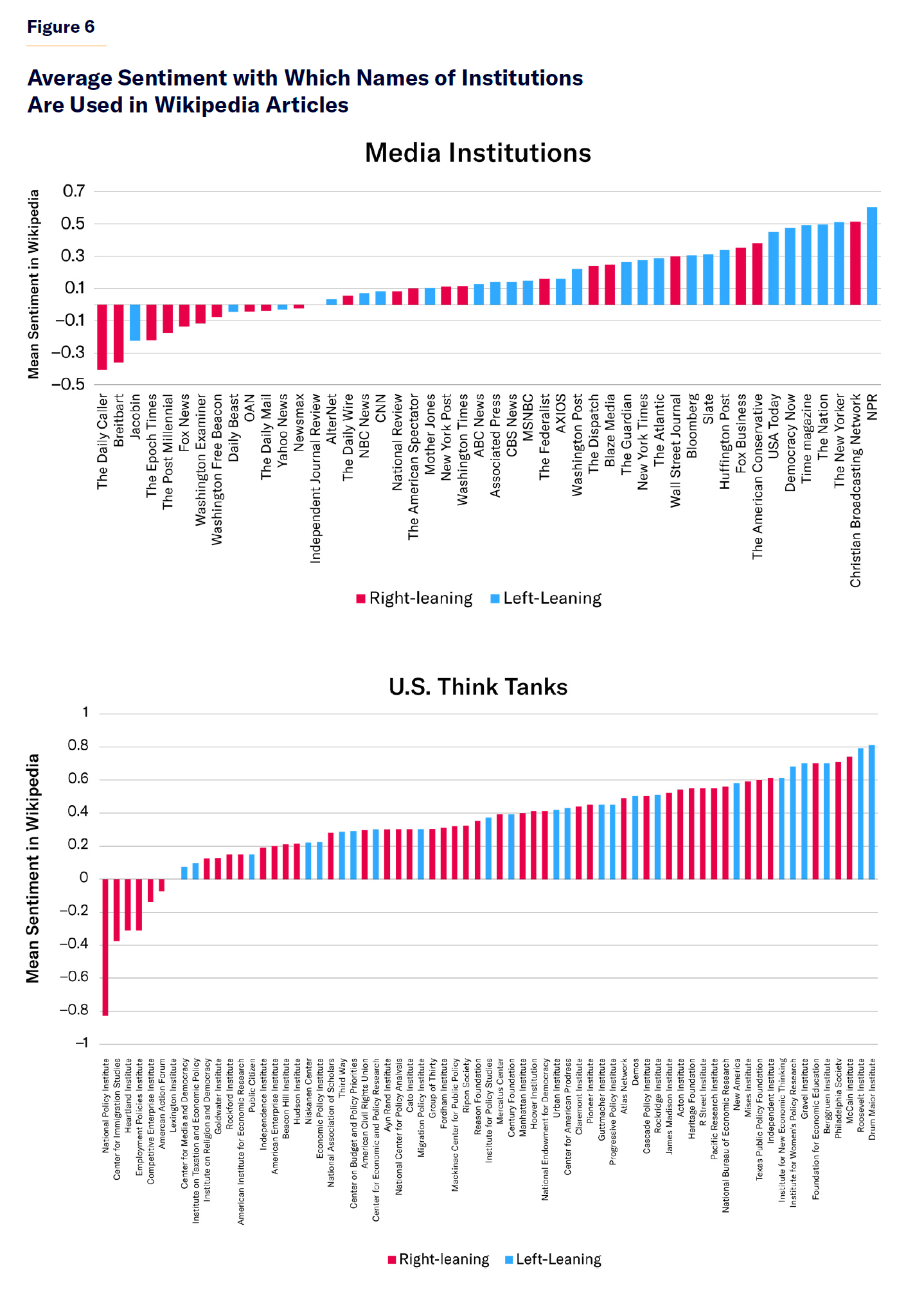
Figure 7 summarizes these trends and applies statistical t-tests for each category of terms to determine whether the difference in sentiment in Wikipedia articles between left-leaning and right-leaning terms is statistically significant. Each category of results that are statistically significant at the 0.01 threshold is marked with an asterisk. However, because the categories vary widely in their number of terms, and said p-values are dependent on the sample size of each test, the p-values alone do not say much about the magnitude of the difference between the two groups. Thus, Figure 7 also provides an estimate of the effect size (Cohen’s d) for the difference between the two political groups in each test. For most of the categories analyzed, there are substantial effect sizes for the differences in the sentiment with which right-leaning and left-leaning terms are used in Wikipedia articles. The t-test for U.K. MPs is borderline-significant, but the effect size is negligible.
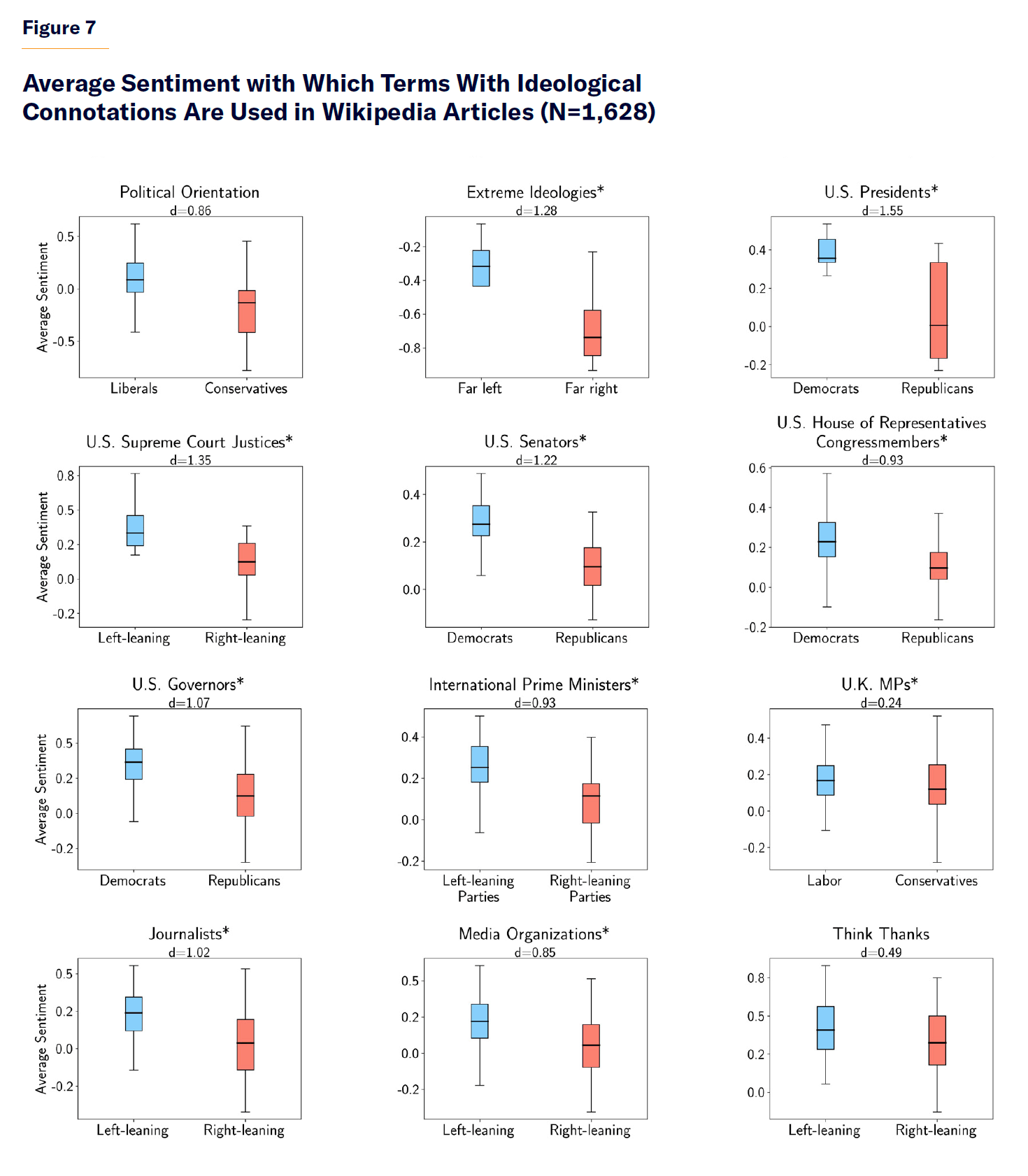
Next, we analyze the average emotion with which these terms with political connotations are used in Wikipedia articles, using Ekman’s six basic emotions (anger, disgust, fear, joy, sadness, and surprise)[11] plus neutral. For ease of visualization, we exclude the emotionally neutral category in the next visualization. Figure 8 shows that, in Wikipedia articles, right-leaning terms are more often associated with the emotional categories of anger and disgust than left-leaning terms. In contrast, left-leaning terms are more often associated with the emotion of joy than right-leaning terms.
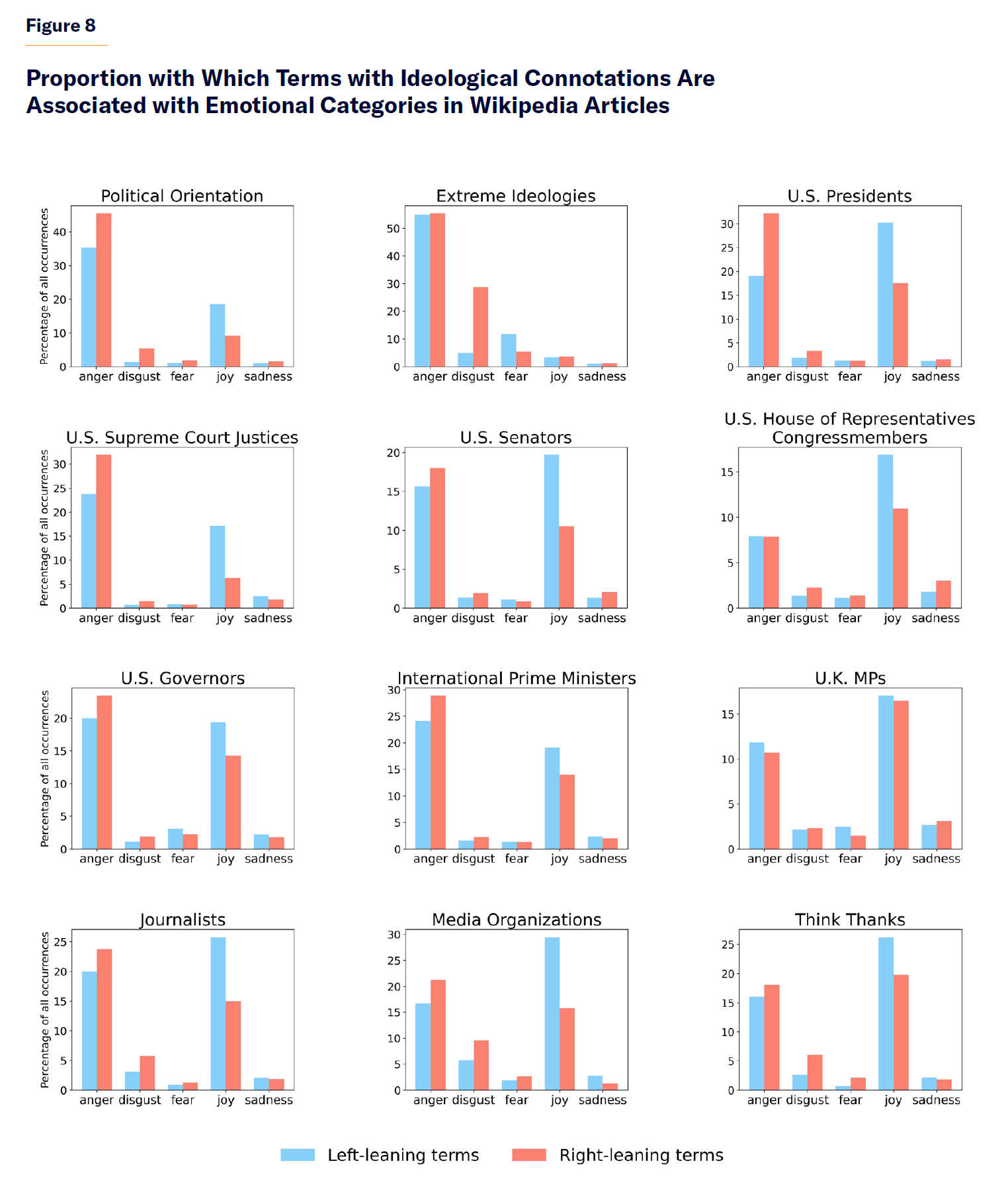
The Potential for Wikipedia Biases to Percolate into Artificial Intelligence Systems
Whether, and to what extent, there is political bias in Wikipedia articles has become increasingly important due to the growing societal influence of AI systems trained with Wikipedia content. Therefore, we investigate next whether the association between left-wing terms and greater positive sentiment in Wikipedia articles can also be seen in OpenAI’s language models. To address this question, we analyze a critical subcomponent—the word embeddings model—of OpenAI’s GPT LLM series.
Word embeddings are a by-product of the pretraining of an LLM. They are mappings from words (or “tokens”) to numerical vector representations that encapsulate the semantic and syntactic loading with which a word or token is used in the corpus of text used to pretrain the LLM.
The embeddings represent words (or, more accurately, tokens, which can be parts of words, entire words, or several words) as vectors in a high-dimensional space. The position of a word in this space relative to others reflects the semantic and syntactic relationships derived from the text corpus on which the model is trained. The embeddings pick up, among other relationships, frequent co-occurrences (associations) between pairs of terms in the corpus. If two terms often co-occur in similar contexts in a corpus of text, they will tend to have more similar embeddings.
For our experiments, we first derive our own word embeddings model from the entirety of Wikipedia articles using the word2vec algorithm.[12] We then estimate the association strengths between our target political terms and a frequently used sentiment lexicon (AFINN).[13] In Figure 9, the left pole of each category subplot represents in vector space an aggregate of the Wikipedia-derived word embeddings for the left-leaning terms in that category; the right pole of each subplot shows the same for right-leaning terms in that category. Positive sentiment terms from the AFINN lexicon are color-coded in green; and negative terms are in red. Measures of the strength of association between our set of target terms and AFINN sentiment terms are derived using cosine similarity between their vector embeddings representations in vector space.
Figure 9 presents histograms for this measure from all the pairwise comparisons between AFINN sentiment terms and the political poles in each category. In most subplots, the green bars (positive sentiment) are skewed to the left pole (representing left-leaning terms), while the red bars (negative sentiment) are skewed to the right pole (right-leaning terms). In our word embeddings model derived from Wikipedia articles, left-leaning terms are typically closer to positive-sentiment terms, while right-leaning terms are closer to negative-sentiment terms. This indicates Wikipedia’s tendency to more often associate positive terms with left-of-center political terms and negative terms with right-of-center political terms.
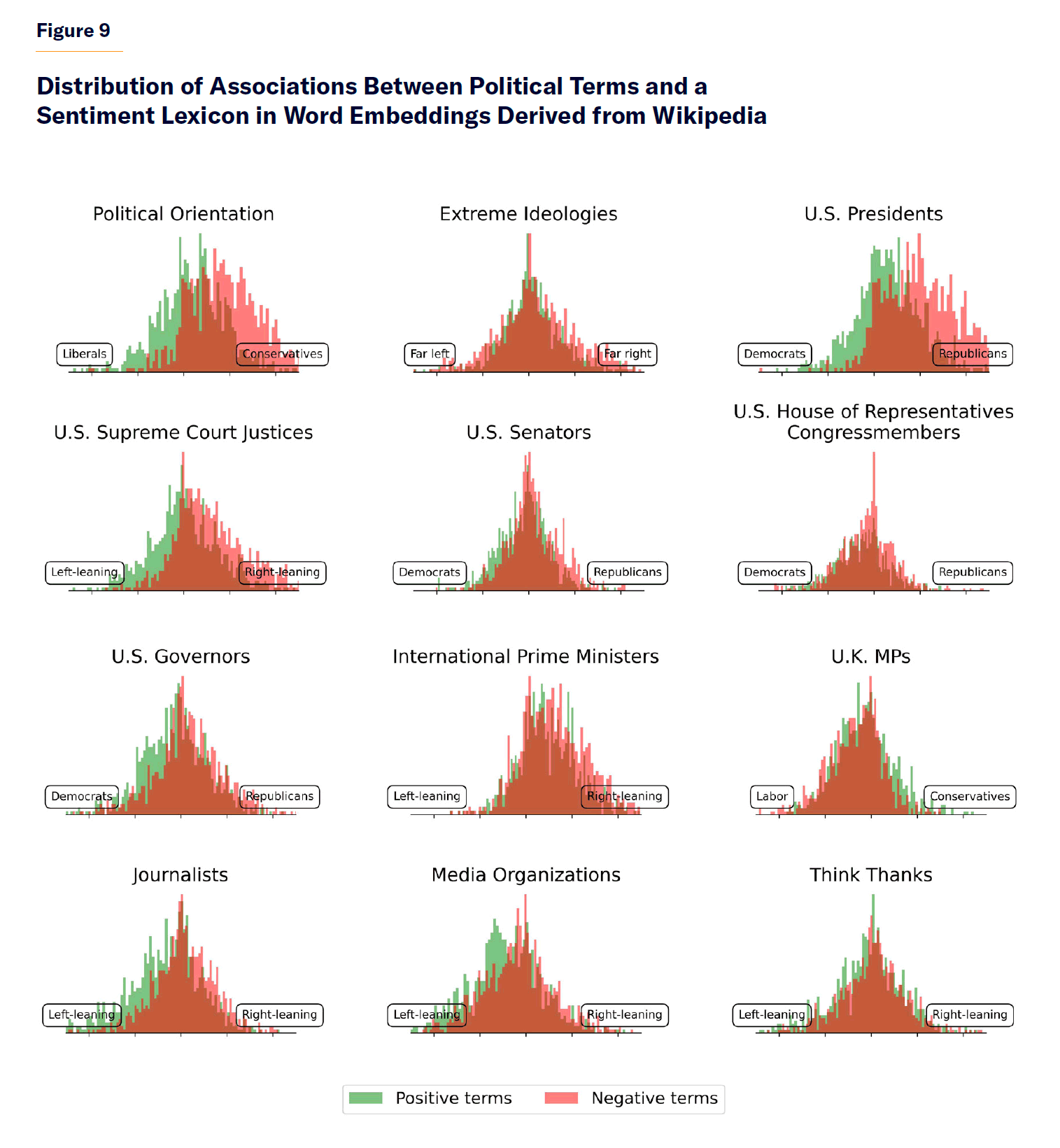
Finally, we analyze the extent to which the same trends just described for word embeddings derived from Wikipedia content are also present in OpenAI embeddings, a critical subcomponent of semantic meaning in the ChatGPT system. The OpenAI embeddings are derived from the secret training corpus used by OpenAI to train their GPT models and which presumably contain Wikipedia content, as in earlier open-source versions of their GPT models series.[14]
Thus, we calculate the strength of the association between our set of target political terms and sentiment terms in word embeddings derived directly from Wikipedia and in word embeddings from OpenAI. We then plot the correlation between the strength of that association in Wikipedia embedding and the strength of that measure in OpenAI embeddings. We find a mild-to-moderate correlation in the strength of association between both sets of embeddings with sentiment terms (Figure 10). Note the positive slope of the relationship between the x and y variables: there is a degree of overlap in the prevailing sentiment associations of political terms in word embeddings derived from Wikipedia content and word embeddings from the OpenAI GPT series. This is not surprising, given that Wikipedia articles are likely a prominent part of OpenAI’s secret corpus of data used to train ChatGPT. But we believe that it was important to document this phenomenon to illustrate the potential for biases embedded in Wikipedia content to percolate into state-of-the-art AI systems.
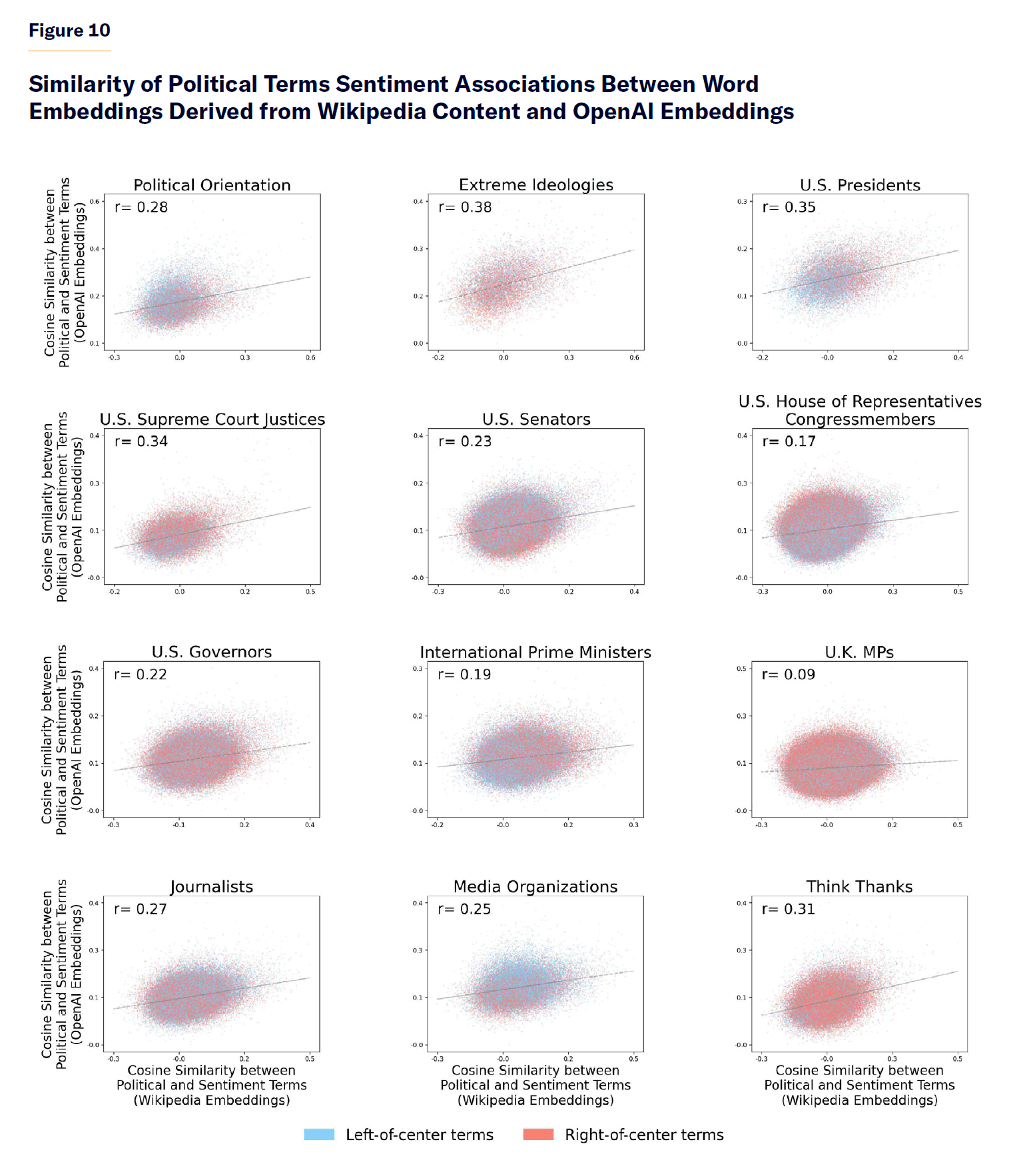
Discussion
A potential criticism of our results’ interpretation might be called the “self-sorting emotional affect hypothesis”: some research has suggested that conservatives themselves are more prone to negative emotions and more sensitive to threats than liberals,[15] which would perhaps explain why terms associated with right-leaning politics are used more often with negative sentiment and emotion in Wikipedia articles; right-wing figures might make statements with more negative sentiment and emotion, which are simply reported in Wikipedia articles. But there are reasons to doubt this hypothesis: other studies have disputed that conservatives are more prone to negative emotions,[16] and conservatives often report higher levels of happiness and life satisfaction than liberals.[17] Additionally, we specifically instructed the machine-learning model to annotate the sentiment with which the entity was being used in the text, thereby trying to avoid incorrect categorization in instances where the target entity might be reported as saying something negative.
A substantial body of literature—albeit with some exceptions—has highlighted a perceived bias in Wikipedia content in favor of left-leaning perspectives. The quantitative findings presented in this report are consistent with that literature, providing further support for the hypothesis of left-leaning bias in Wikipedia content.
Our results on the correlation between sentiment associations in Wikipedia-derived word embeddings and those from OpenAI suggest that Wikipedia biases might be percolating into state-of-the-art AI systems. Of course, correlation does not equate to causation; but in this instance, the evidence hints at a directional influence. We can safely exclude the possibility of reverse causality (biases from OpenAI embeddings influencing Wikipedia content in 2022), given that Wikipedia’s existence predates OpenAI’s AI models and robust coherent text generation by language models is a relatively new phenomenon. Furthermore, as of the writing of this report, we are unaware of Wikipedia using generative AI to create English textual content. Hence, any mechanism attempting to explain the correlation using reverse causality seems convoluted or implausible.
The specifics of the data sets used for training the latest ChatGPT (GPT-4) remain undisclosed.[18] But OpenAI has previously acknowledged using Wikipedia content in the training data sets of earlier GPT models (like GPT-3), so it is likely that Wikipedia still plays a significant role in the training material for the latest models.[19] Other popular LLMs, such as the open-source LLaMA series from Meta or PaLM from Google, have openly described their usage of Wikipedia in their training corpora.[20] Thus, there is a causal mechanism to explain how Wikipedia biases would infiltrate into the memory parameters of LLM models. We cannot, however, exclude the possibility of a hidden variable influencing both Wikipedia content and LLMs simultaneously. We also note that, paradoxically, pretrained base LLMs do not appear to reveal strong ideological preferences when responding to political orientation tests; but they do show strong ideological preferences after fine-tuning and Reinforcement Learning from Human Feedback (RLHF). However, a number of limitations about properly measuring political bias in base models have been identified.[21]
Nevertheless, the available evidence suggests that the most likely direction of causality is that Wikipedia content is influencing the parameters of LLMs.
Conclusion
Using a novel methodology—computational content analysis using a Large Language Model for content annotation—this report measures the sentiment and emotion with which political terms are used in Wikipedia articles, finding that Wikipedia entries are more likely to attach negative sentiment to terms associated with a right-leaning political orientation than to left-leaning terms. Moreover, terms that suggest a right-wing political stance are more frequently connected with emotions of anger and disgust than those that suggest a left-wing stance. Conversely, terms associated with left-leaning ideology are more frequently linked with the emotion of joy than are right-leaning terms.
Our findings suggest that Wikipedia is not entirely living up to its neutral point of view policy, which aims to ensure that content is presented in an unbiased and balanced manner. Our analysis also shows that these biases in Wikipedia might already be infiltrating and shaping widely used AI systems. Given Wikipedia’s status as one of the most visited sites globally, the implications of these political biases—both in influencing public opinion and shaping AI technologies—are concerning.
The goal of this report is to foster awareness and encourage a reevaluation of content standards and policies to safeguard the integrity of the information on Wikipedia being consumed by both human readers and AI systems. To address political bias, Wikipedia’s editors could benefit from advanced computational tools designed to highlight potentially biased content. Additionally, collaborative tools similar to X’s Community Notes could also be instrumental in identifying and correcting bias. While acknowledging Wikipedia’s immense contributions to open knowledge, we see its journey towards complete impartiality as ongoing.
Appendix: Methods
Wikipedia corpus and preprocessing
We use an English Wikipedia dump file from 2022 (enwiki-20221001-pages-articles-multistream. xml, size 89 GB) for our analysis. We parse the raw Wikipedia content in the xml file to extract just the text contained in Wikipedia articles. We filter out from the dump file non-article content (such as metadata and non-textual content). The final size of the file containing just text from Wikipedia English articles is 23.6 GB.
We then locate in the cleaned text all paragraphs containing any of our set of 1,652 target terms analyzed (1,628 of which belong to our set of terms with political connotations and the remaining 24 which are used for sanity check purposes due to their overwhelming positive/negative connotations, see Figure 1 for illustration). This produces 3.8 million paragraphs containing any of our set of target terms.
Target-term sources
To reduce the degrees of freedom of our analysis, we mostly use external sources of terms to conceptualize a political category into left- and right-leaning terms, as well as to choose the set of terms to include in each category.
For the category of left and right political extremism, we use a reduced set of terms from the existing academic literature.[22]
For the U.S. presidents category, we use the most recent U.S. presidents since Jimmy Carter.
For the Western countries’ prime-minister category, we use Wikipedia for a list of all the prime ministers since 2000 in prominent Western countries: the most populous countries in continental Europe (Germany, France, Italy, Spain, and Poland) plus Canada, the U.K., Australia, and New Zealand.
For the U.S. Supreme Court justices list, we use Wikipedia’s article on the ideological leanings of the most recent U.S. Supreme Court justices.[23]
For the members of the U.S. House of Representatives and the U.S. Senate, we use the list of members of the 117th United States Congress from Wikipedia.[24]
For the list of state governors, we use Wikipedia’s page on the topic and select those who were in office as of 2022, since that is the date of the Wikipedia dump file we used in our analysis.[25] We use 2022—rather than the year of the writing of this report, 2024—in order to allow for solidification of content, as opposed to using more recent governors who may not have been in office long enough for sufficient information about them to have accumulated on Wikipedia.
For the list of U.K. MPs, we use the Wikipedia list of U.K. MPs elected in the 2019 U.K. general election.[26]
For the list of U.S.-based journalists, we use a list of politically aligned U.S.-based journalists from Politico.[27]
For the list of U.S. news media organizations, we use the AllSides ranking of political bias in news media organizations as well as the complete list of news media organizations in its Media Bias Chart.[28]
For the list of U.S.-based think tanks and their political leanings, we use Wikipedia’s list on the topic as well as their political bias/affiliation according to Wikipedia.[29]
Sentiment annotation and analysis
We use automated sentiment annotation in our analysis using a modern LLM, gpt-3.5-turbo. The decision to use automated annotation is based on recent evidence showing how top-of-the-rank LLMs outperform crowd workers for text-annotation tasks such as stance detection.[30] The set of system and user prompts used to generate the sentiment annotations are provided in electronic form in the open access data repository associated with this work (see link below).
We map the sentiment labels (positive, neutral, negative) to numerical values (1, 0, –1) to estimate average sentiment of mentions of a term in Wikipedia content.
To keep the costs of our analysis under control, we used a sample of paragraphs containing each of our target terms. We targeted 200 sentiment annotations per target term to obtain an estimate of the sentiment with which the term is used in a representative sample of Wikipedia paragraphs containing the term. We also repeated the analysis with a different sample of 200 instances of each target term analyzed with similar results to what has been reported above. Sometimes, the number of instances of low-frequency terms in Wikipedia content is lower than 200. For our visualizations in Figures 1–6, we used a minimum threshold for inclusion of at least 20 Wikipedia text-occurrence annotations for each term. In total, we generated 175,205 sentiment annotations for the set of 1,652 target terms analyzed. That is an average of 106 sentiment annotations for each term analyzed.
Emotion annotation and analysis
For the emotion annotation analysis, we used a similar process to the one described above for sentiment analysis. We use Ekman’s six basic emotions (anger, disgust, fear, joy, sadness, surprise) plus neutral to automatically label the emotion with which our target terms are used in Wikipedia paragraphs. The inclusion of the neutral category is to provide the LLM model with the possibility of not labeling a particular instance of a target term with a loaded emotion, since many occurrences of a target term might indeed be emotionally neutral. We exclude the neutral category in Figure 8 for ease of visualization and to focus the results on Ekman’s six basic emotions.
In total, we generated 175,283 emotional annotations for our 1,652 set of target terms analyzed. That is an average of 106 emotion annotations for each term analyzed.
Open access data
The set of 1,652 target terms used in our analysis, the sample of Wikipedia paragraphs where they occur (as of Wikipedia 2022 dump enwiki-20221001-pages-articles-multistream), and their sentiment and emotion annotations are provided in the following open-access repository:
https://doi.org/10.5281/zenodo.10775984Deriving word embeddings from Wikipedia content
We use Gensim’s implementation of the word2vec algorithm to derive word embeddings from Wikipedia textual content.[31] We preprocess the Wikipedia corpus to annotate n-grams from our set of target terms with an underscore marker (e.g., “Barack Obama” becomes “Barack_Obama”). The hyper-parameters we used during word2vec training were the continuous bag-of-words method, vector size=300, window size=10, minimum number of occurrences for inclusion into the model=10, negative sampling=10, down sampling=0.001, number of training epochs through the corpus=2.
For evaluation of accuracy, we used the commonly used wordsim353.tsv data set for similarity evaluation, obtaining a 0.66% Pearson correlation with human raters (0.68 Spearman r). We also used the word analogies evaluation data set released by Google when it published word2vec, obtaining 76% accuracy. Both results are consistent with the metrics reported by Google when it published its word2vec embeddings model trained on the Google News data set.
AFINN Lexicon
We use the AFINN sentiment lexicon (N=2,477) in our analysis. The lexicon is unbalanced, as it contains more negative terms than positive terms. We balance the lexicon by taking 878 random terms from each sentiment category (positive and negative) for a final balanced lexicon of size N=1,756.
OpenAI word embeddings
We retrieved OpenAI text embeddings using OpenAI’s API. In our analysis above, we report results for the “text-embedding-3-large” embeddings model, but similar results are obtained when using the “text-embedding-3-small” embeddings model.
Endnotes
Photo by Artur Widak/NurPhoto via Getty Images
Are you interested in supporting the Manhattan Institute’s public-interest research and journalism? As a 501(c)(3) nonprofit, donations in support of MI and its scholars’ work are fully tax-deductible as provided by law (EIN #13-2912529).