Do Black Newborns Fare Better with Black Doctors? The Limits of Measuring Racial Concordance
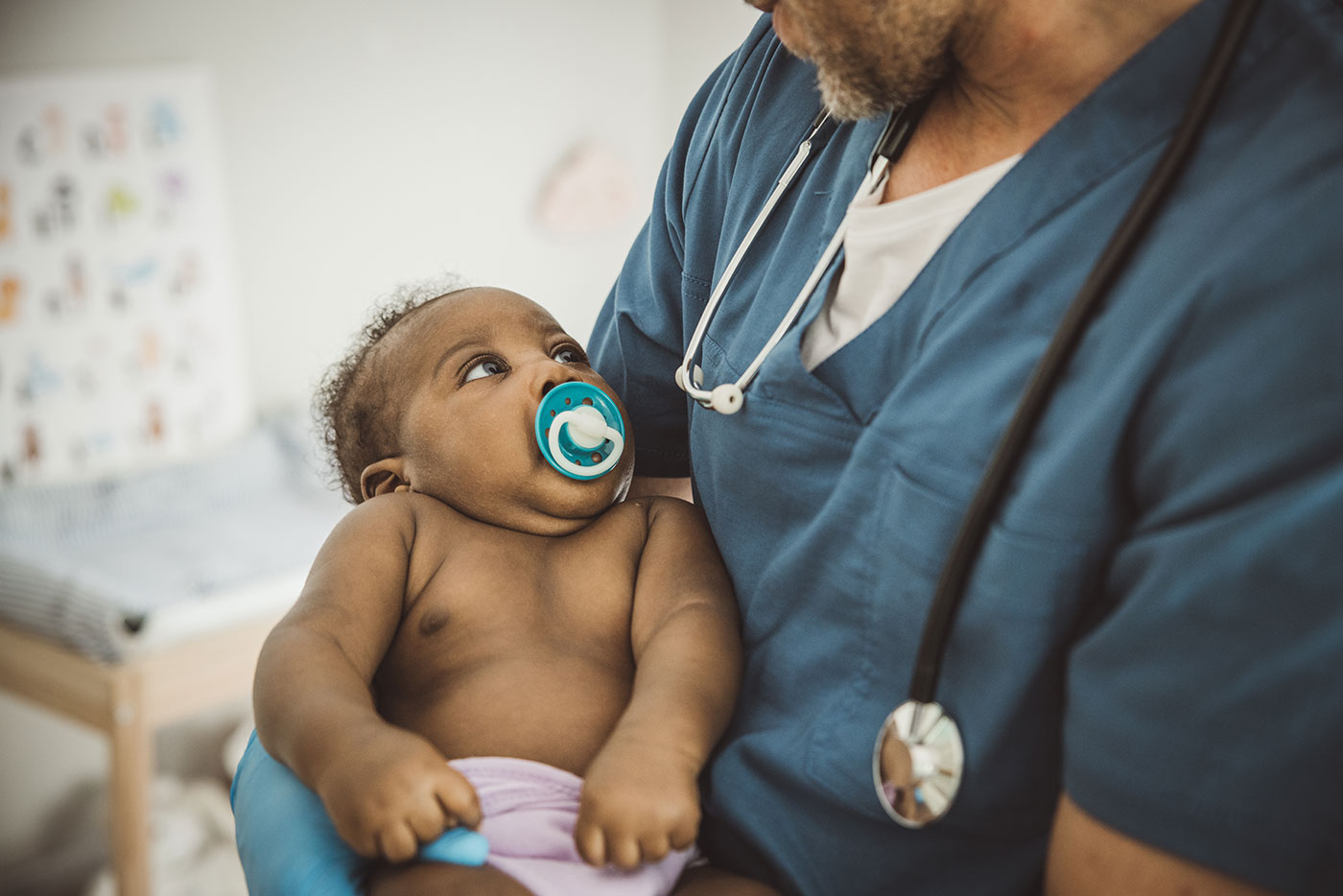
Introduction
In August 2020, the Proceedings of the National Academy of Sciences (PNAS) published an influential academic paper with a striking empirical finding.[1] Using data from Florida hospitals over three decades, the study found that black babies were less likely to die if cared for by black physicians, instead of white physicians, after birth.
In the Florida data set, about eight in every 1,000 black newborns died before leaving the hospital, as compared with only three in every 1,000 white newborns—reflecting the tragic, nationwide racial gap in neonatal mortality. Being cared for by a black doctor, however, appeared to reduce a black newborn’s chance of death. In the raw data, the death rate for black babies seen by black doctors was only four in 1,000. Even in a statistical model that controlled for the newborns’ health conditions and other factors, being seen by a black doctor appeared to reduce black newborns’ mortality by about 1.3 per 1,000.
The article created a stir—unsurprising, given the importance of the topic and the findings’ ramifications—for patients’ choices, for hospitals’ practices, and for the debate over affirmative action. Media outlets including the Washington Post and CNN covered the paper. In 2023, drawing from an amicus brief filed by the Association of American Medical Colleges, Justice Ketanji Brown Jackson cited the finding in her dissent in Students for Fair Admissions v. Harvard, which addressed the legality of racial preferences in higher education.[2]
In modern social science, however, there are always many ways of analyzing the same data—and sometimes, important details go unnoticed even in peer-reviewed studies. We obtained the same Florida hospital data set with the goal of replicating the study’s findings and running additional models that might help determine just how robust those conclusions are.
We discovered an important repercussion of the way the authors controlled for the infants’ health conditions. The analysis accounts for the 65 health conditions that are most common in the data set, using “diagnosis codes” that are reported for each patient—effectively comparing how the race of the doctor and the race of the newborn interact among newborns who are “equally healthy” in terms of those top 65 “comorbidities.” However, the top 65 comorbidities do not include a single indicator for whether the newborn has a very low birth weight, which is a crucial determinant of infant mortality.[3]
Controlling for very low birth weight—i.e., comparing newborns within the same weight class—eliminates the racial concordance result in the most detailed statistical models. This happens because black doctors disproportionately care for black newborns with healthy birth weights. Conversely, white doctors care for a disproportionately large share of the black babies most at risk, those who have a very low birth weight.
PNAS published our academic paper outlining these results on September 16, 2024.[4] This brief is a reader-friendly summary of how we approached the data, what we found, and what this episode can teach the public about newborn mortality and empirical social science more generally.
The Original Study
The 2020 study relies on hospital discharge records from Florida. To get around some data limitations, the original research team examined births between 1992 through the third quarter of 2015, added the doctors’ races to the hospital admissions data by looking up their photos online, and restricted the data to cases where both the doctor and patient were either white or black.
A striking pattern appears in the raw data. About 0.3% of white newborns died before being discharged from the hospital, regardless of whether their doctor was white or black. For black newborns, however, mortality varied dramatically by physician race: 0.9% for those cared for by white physicians after birth, but just 0.4% for those with black physicians. This reduction, of 0.5 percentage points, can be called the “racial concordance effect,” or the statistical interaction of doctor and patient race. It raises the possibility that black doctors are more skillful in some general sense in treating black newborns.
However, different births present different risks, different doctors see different types of patients, and medical practice can change over time or differ across hospitals. Therefore, other explanations are also possible—for example, there may be notable differences in the care or facilities available in different hospitals. To address such possibilities, the authors added numerous control variables to their models to help determine if the racial concordance effect persisted even after comparing black and white newborns born in relatively similar situations.
These variables included the type of insurance covering the newborn, the year and quarter the birth took place, and the 65 most common health conditions that affect newborns and are reported in the data (as well as whether the baby was born outside the hospital before being brought in). They also included a separate control variable for each combination of hospital and year (to reflect that hospitals have different treatment practices that might change over time) and a separate control variable for each individual physician (to reflect that different physicians will have different skills and see different mixes of patients). Unlike the raw mortality rates discussed above, a model that includes these variables measures differences in mortality among newborns who are similar on all these other factors.
Yet even with differences in all these variables accounted for, black patients seen by black doctors had a lower mortality rate than those seen by white doctors, by 0.13 percentage points. Since the mortality rate for black newborns is about 0.8%, this concordance effect implies that black doctors caring for black newborns would reduce the mortality rate of black newborns by about one-sixth.
The authors laid out some important caveats, in the paper itself and in an online appendix. First, they were examining real-world observational data, not a randomized scientific experiment. In many cases, newborns are assigned to whatever doctor happens to be available at the hospital. Given the length of pregnancy, however, parents have plenty of time to pick their own doctor if they want to, a decidedly nonrandom process. Second, the data identify only the attending physician, not the broader team that helps care for the newborn. Finally, while the models include variables that can help account for nonrandom differences in the types of newborns assigned to white and black doctors—and these controls reduced the estimate of the concordance effect from 0.5 percentage points to just above 0.1—it is possible that other, unincluded variables could reduce the effect further or eliminate it entirely.
Our Analysis
We obtained the data from the same source, and the original authors generously provided us with the physician-race variable that they had created. Our agreement with the Florida Agency for Health Care Administration requires us to state: the “Agency specifically disclaims responsibility for any analysis, interpretations, or conclusions that may be created as a result of the limited data set.”
With guidance from the data construction description in the original study and correspondence with the lead author, we were able to replicate the findings quite closely (though not exactly, as we did not have access to the authors’ full code). But to extend the analysis, we ultimately homed in on the crucial role played by birth weight.
Table S1 of the original study lists the 65 health conditions that the authors controlled for.[5] These conditions range from the basic type of birth (such as a single C-section birth in a hospital), to scalp injuries, to cardiac problems. Numerous birth-weight diagnoses are in this table—but none indicates a birth weight below 1,500 grams (3 pounds, 5 ounces), which health authorities classify as “very low birth weight.” CDC data indicate that while just over 1% of babies are born with very low birth weight nationwide,[6] they account for about two-thirds of all neonatal mortality,[7] i.e., death within the first 28 days of life. The diagnosis of very low birth weight varies dramatically by race, with only 1% of white newborns but about 3% of black newborns weighing less than 1,500 grams nationally.[8] Very low birth weight is thus a strong candidate for an important omitted variable.
For simplicity, in Table 1 we start by reporting the racial concordance effect from the most complete statistical model presented in the original study.[9] As noted earlier, the original study found that a black baby attended by a black physician has a 0.13-percentage-point lower probability of mortality than a black baby attended by a white physician. We then replicate the finding using our data, which gives an extremely similar result. Next, we show that the 65 health conditions included in the original study make little difference to the ultimate finding; removing them from the model entirely (while leaving the other control variables) still leaves the concordance effect at roughly 0.13 percentage points.
However, adding a single variable indicating whether the child has a very low birth weight drastically reduces this number and renders it statistically insignificant (i.e., the margin of error around the estimated concordance effect is large enough that one could not reject the hypothesis that the true effect is zero). This single variable improves the model itself, increasing the percentage of variance explained (i.e., the R-squared) from 16% in our replication of their full model to 21%. In short, this variable explains the newborns’ mortality better than the top 65 diagnoses combined and renders the concordance effect insignificant.
Table 1
Racial Concordance Effect on Assorted Models
Model | Concordance Effect (in Percentage Points) |
Original result | –0.129* |
Replication (top 65 comorbidities + controls for insurance, quarter-year and hospital-year combinations, and physician) | –0.136* |
Remove top 65 entirely | –0.131* |
Replace entire top 65 with single control for under 1,500 grams birth weight | –0.033 |
Replace single weight control with detailed controls for under 1,500 grams birth weight | –0.014 |
Include all 4,050 available comorbidities | –0.027 |
Include all combinations of comorbidities | –0.000 |
* p < 0.1, n = 1,863,837
Including separate controls for each type of very-low-birth-weight diagnosis—there are 30 in total, with specific weight ranges and classifications of the newborn’s condition (such as “‘Light-for-dates’ without mention of fetal malnutrition, 1,000–1,249 grams”)—boosts the R-squared even more (to nearly 40%) and still suggests no statistically significant concordance effect.
We also ran models that include all diagnoses that appear in the data, rather than limiting the controls to the top 65 comorbidities or to the very-low-birth-weight condition.[10] The Florida hospital data report up to 31 different diagnoses for each newborn, and each of these diagnoses can indicate the presence of hundreds of potential issues with the birth. As a result, there are actually 4,050 different diagnoses in our sample of newborns. Controlling for the presence of each of these diagnoses still reveals no concordance effect.
Of course, the key question is why simply controlling for differences in very low birth weight among newborns eliminates the concordance effect. Statistically, it is not enough that this condition is a strong predictor of mortality, or even that black newborns are more likely to suffer from low birth weight. Among black newborns, there must be some difference in birth weight between those cared for by white versus black doctors that is not present among white newborns who see doctors of different races.
To investigate, we collapsed the numerous birth-weight codes into simple weight ranges. Most newborns do not have a diagnosis requiring their weight to be recorded in this data set, so the most common classification in the Florida data (containing about 92% of the sample) is “No Code.” Then we separated the data by the doctors’ and patients’ races. Figure 1 shows the results in two ways.
As shown in the left panel, about 10% of white babies in the sample have black doctors, and this fraction changes little with the newborns’ weight. Meanwhile, healthy-weight black babies are much more likely to have black doctors—as might happen for any number of reasons, ranging from parents choosing racially concordant doctors for their infants, to nonrandom matching by hospital staff. (We are not physicians or health-care specialists and do not want to speculate.)
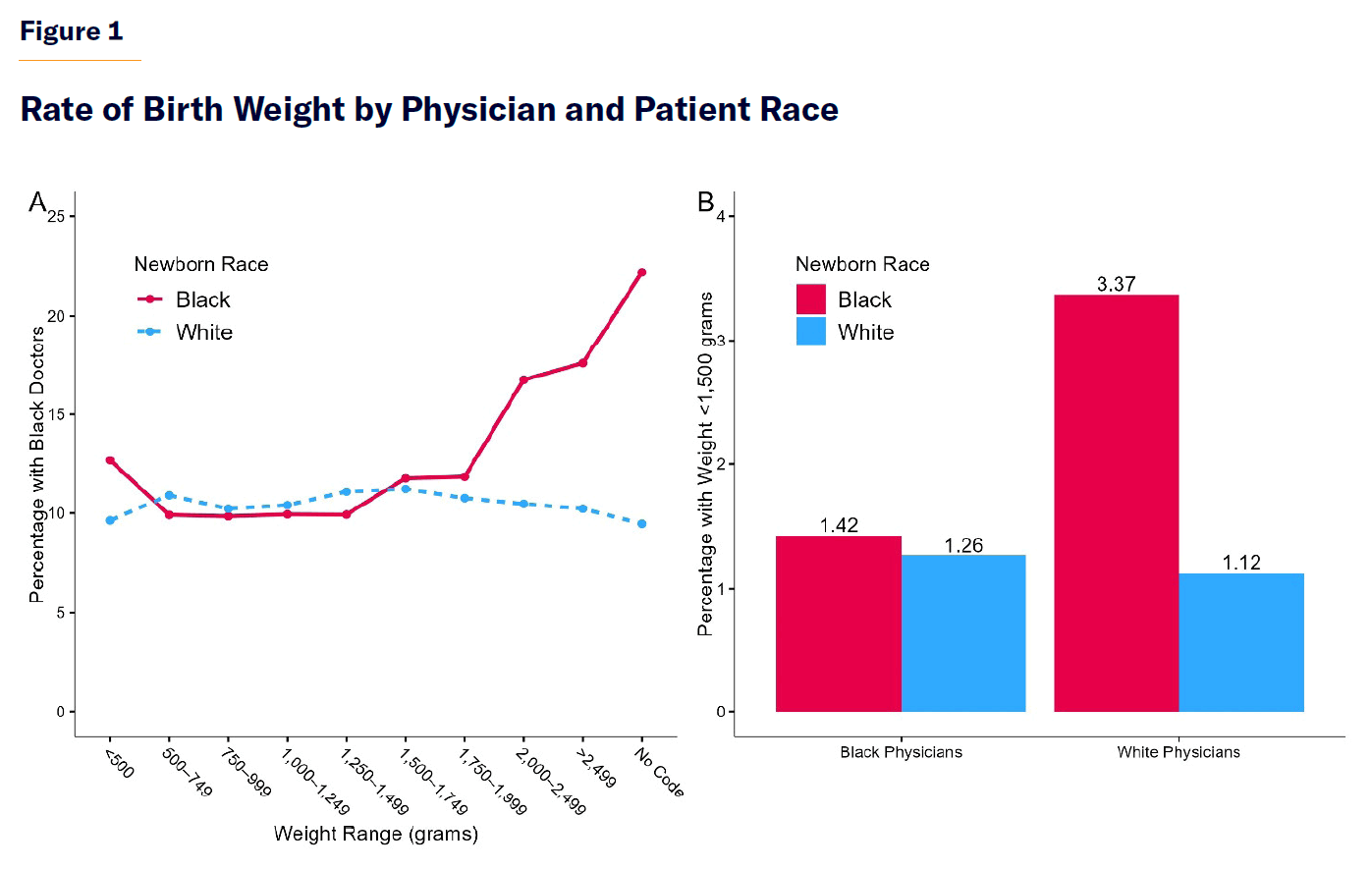
Crucially, black newborns with the lowest birth weights are not strongly sorted toward black doctors. Like white babies, they have black doctors only about a tenth of the time. I.e., whatever mechanism directs black newborns to black doctors in normal situations seems to operate differently when babies have the very serious diagnosis of a very low birth weight.
The right panel of the figure looks at the same breakdown through a different lens. Because black doctors experience such a strong skew toward black infants with healthy weights, the racial birth-weight gap appears mostly among newborns attended to by white doctors. White doctors are disproportionately more likely to attend black newborns with very low birth weights, a condition closely linked with newborn mortality. In a model that fails to control for these birth weights directly, white doctor / black patient combinations will thus appear particularly lethal.
What About the “Main Effects” of Doctor and Patient Race?
We have focused on the interaction of doctor and patient race—which measures whether something special happens when a black doctor and a black patient come together—because this is where the potential racial concordance effect appears in the statistical model. However, the “main effects” of the doctor’s and patient’s race are also of interest: Are mortality rates higher for babies attended by black doctors than for babies attended by white doctors, even after controlling for comorbidities and other variables? Similarly, are adjusted mortality rates higher for black newborns than for white newborns? In this section, we answer these questions, providing additional information from our models that is not discussed in our PNAS paper.
The main effect of the doctor’s race tells us whether white or black doctors tend to have better baseline mortality outcomes, after accounting for the other variables in the model (and abstracting from any potential racial concordance effect). Specifically, it represents the difference in mortality that white babies experience when they are cared for by black instead of white doctors. Whether black or white doctors have better mortality outcomes is obviously relevant to debates over affirmative action and the attendant concerns about physician performance.
The estimated models reported in the original study showed little sign of any link between the race of the doctor and the overall mortality rate. Our models suggest the same thing. Black and white doctors tend to have about the same mortality outcomes when other variables are held equal—when they work in the same hospitals at the same time, treat similar types of patients, etc. In fact, we never find any statistically significant difference between the mortality rates of babies attended by white and black doctors, regardless of the set of controls we use.
Table 2 reports the effect of having a black doctor on the mortality rate of white babies in the most complete models where this effect is measurable.[11]
Table 2
Difference in Baseline Mortality Rate Between Newborns Attended by a Black and a White Doctor
Model | Main Effect (in Percentage Points) |
Unadjusted mortality gap | –0.010 |
Mortality gap adjusted for type of insurance, quarter-year, and hospital of birth and: | 0.063 |
Top 65 comorbidities | 0.046 |
Very low birth weight (under 1,500 grams) | 0.003 |
Detailed controls for very low birth weight | 0.023 |
All 4,050 available comorbidities | 0.022 |
The key implication of Table 2 is that the race of the doctor never has a statistically significant effect on the mortality rate of white newborns. Moreover, the magnitude of the measured effect tends to cluster near zero.
Next, regarding the causes of the very large racial gap in newborn mortality, the raw data summarized in Figure 2 suggest that birth weight is extremely important. Black infants have strikingly similar mortality to white infants in the same weight class. Infants of both races have mortality rates above 80% when they weigh 500 grams or less, and mortality rates of 1% or lower if they weigh at least 1,750 grams.
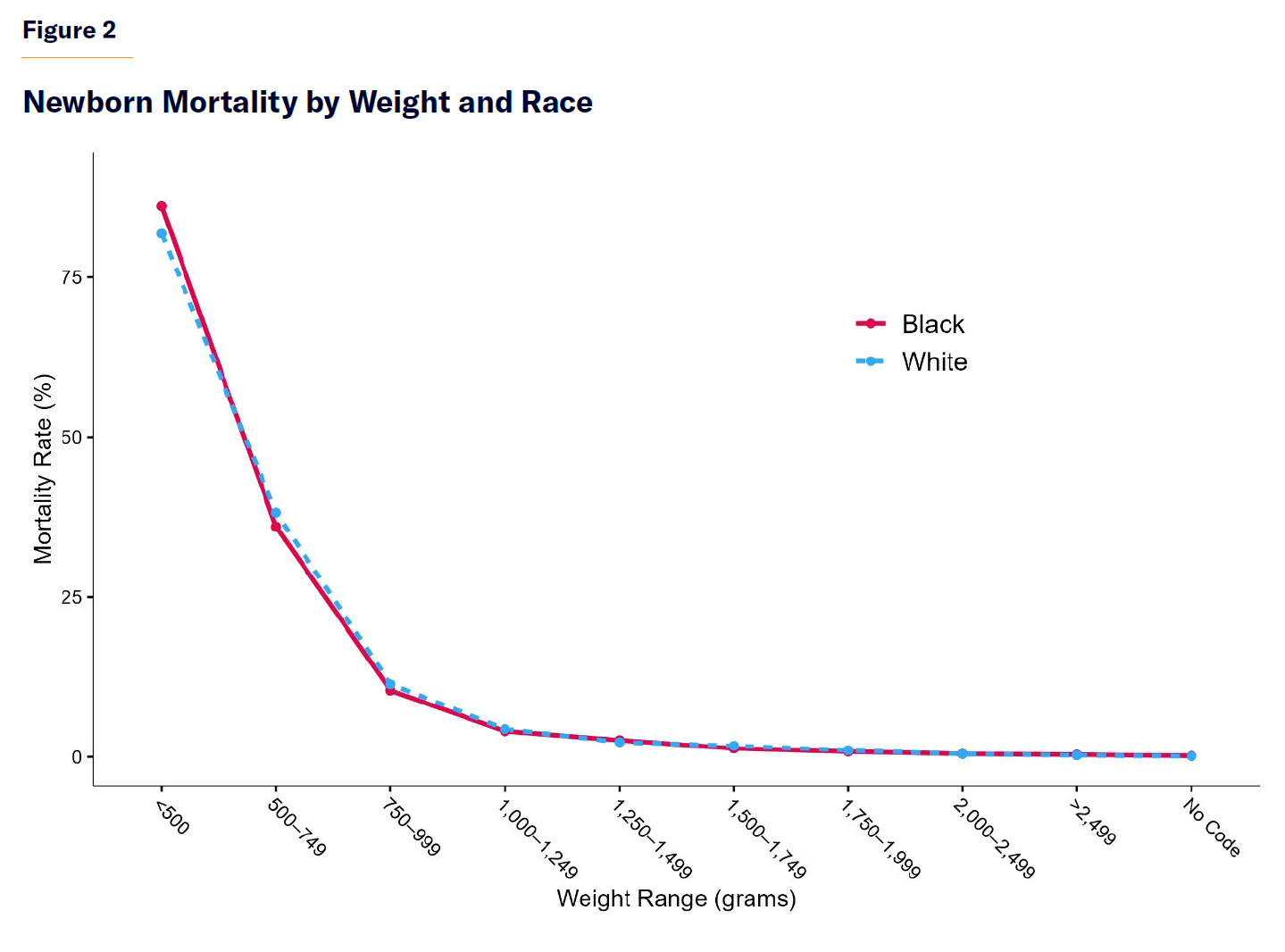
Table 3 shows that adding the single variable indicating a very low birth weight to the basic model (which also controls for all the other variables discussed above) reduces the excess mortality rate of black newborns by about 80%. Moreover, having more detailed controls for the very low birth weight diagnosis or simply controlling for all 4,050 potential comorbidities reported in the data makes the difference between the mortality rate of black and white newborns statistically insignificant.
Table 3
Difference Between Baseline Mortality Rates of Black and White Newborns
Model | Main Effect (in Percentage Points) |
Unadjusted mortality gap | 0.605 |
Mortality gap adjusted for type of insurance, quarter-year, hospital of birth, and physician: | 0.300 |
Adds top 65 comorbidities | 0.339 |
Single control for under 1,500 grams birth weight | 0.114 |
Detailed controls for under 1,500 grams birth weight | 0.020 |
All available comorbidities | 0.018 |
In short, our analysis suggests that the potential for improving neonatal outcomes for black infants lies more in reducing the incidence of newborns born with very low birth weights (and in improving care for those infants) than in the color of their doctors’ skin. Fortunately, care for premature infants has improved rapidly in recent years—though not all hospitals routinely do, or are equipped to do, everything that is possible.[12] Expanding these treatments is thus a promising option for reform.
Limitations and Future Research
Whether the question is racial concordance or the overall effects of doctor and patient race, it is worth stressing how limited our findings are. They are based on data from a single state, ending nearly a decade ago—and involve a specific type of doctor–patient relationship where we might not expect concordance to matter.
Recall that we, like the authors of the original study, are looking at the attending physician who cares for the baby after birth. In the Florida data, the mothers and newborns are recorded as separate patients, and it is not possible to match a mother to her own child in the anonymized records provided to researchers. Thus, it is not possible to, for instance, measure the relationship between the baby’s outcome and the race of his mother’s ob-gyn—which is where we might expect to find a concordance effect if, say, black mothers trusted black doctors more and were more willing to follow their advice in ways that affect the infant’s health.[13]
At the time of birth, many key risk factors have already been established, and especially in complex cases, an entire team of health-care professionals, possibly of diverse races, may begin to care for the baby. Even if concordance effects are a real phenomenon, this is not an ideal setting for them to manifest themselves, or for researchers to accurately measure them.
Ultimately, although we believe skepticism is warranted regarding claims in this contentious area, this is a topic that deserves more study with different data sets, with a special focus on scenarios where physicians and patients are matched randomly. A meaningful concordance effect may exist in certain circumstances, such as when patients’ adherence to a doctor’s advice is key and trust is compromised across racial lines. Whatever the ultimate truth is, patients and health professionals alike should know it.
Conclusion
Our exercise provides two general lessons about the nature of deriving and interpreting empirical results in social science. On the one hand, it shows that consumers of social science must be aware that findings can change with (seemingly minor) technical tweaks to statistical models. In this context, the apparently strong benefits of matching black newborns with black doctors became unmeasurably small after controlling for one single factor, indicating whether a newborn was born weighing less than 1,500 grams.
On the other hand, we were able to replicate and adjust the study’s models because the original authors generously provided their data on the race of physicians, answered our questions, and offered helpful feedback on an early draft of our paper—norms that have become much stronger in recent years, thanks to the “replication crisis” and related scandals. An equally important lesson, then, is that science has the capacity for self-correction, and scientists can facilitate this by being open with their methods and data.
Appendix: Concordance Without Comorbidities?
In addition to the main findings we have focused on, the original study offered several analyses of subsamples of the data. One such analysis is worth briefly discussing here: the authors report finding a concordance effect in a sample of newborns with zero comorbidities, similar in size to the overall effect (–0.11) though not statistically significant. One might reasonably ask how this happened if, as we claim, birth-weight comorbidities are the key driver of the racial concordance effect.
The explanation is that, per the original study, the researchers “split the sample based on whether or not the newborn is diagnosed with at least one of the 65 comorbidities included in the set of controls.”[14] I.e., like the primary analysis, these additional regressions ignore rarer conditions such as diagnoses of very low birth weight. A patient may have a dangerously low birth weight and still be considered to have zero comorbidities.
This part of the original analysis was somewhat more difficult to replicate. If one were to split the same based on whether patients had literally any of the top 65 most common comorbidities, one would hardly split the sample at all. These diagnosis codes include basic birth categorizations—such as V30.00, for “Single liveborn, born in hospital, delivered without mention of cesarean section”—and very few of the newborns have none of the 65 comorbidities. From the paper’s reported sample size (546,516) and the regression code that the authors provided, we infer that the authors specifically exempted V30.00 from being considered a comorbidity when splitting the sample. When we follow this approach, we obtain a sample size for the zero-comorbidity sample that is close to their sample size.
At any rate, adding the controls of very low birth weight to this model once again reduces the concordance effect to a magnitude that is numerically and statistically near zero.
Table A1
Concordance in Sample with No Top 65 Comorbidities (Excepting V30.00)
Model | Concordance Effect (in Percentage Points) |
Original Result | –0.110 |
Replication of full model | –0.147 |
Single control for under 1,500 grams birth weight | 0.009 |
Detailed controls for under 1,500 grams birth weight | 0.013 |
Endnotes
Photo: svetikd / iStock / Getty Images Plus
Are you interested in supporting the Manhattan Institute’s public-interest research and journalism? As a 501(c)(3) nonprofit, donations in support of MI and its scholars’ work are fully tax-deductible as provided by law (EIN #13-2912529).